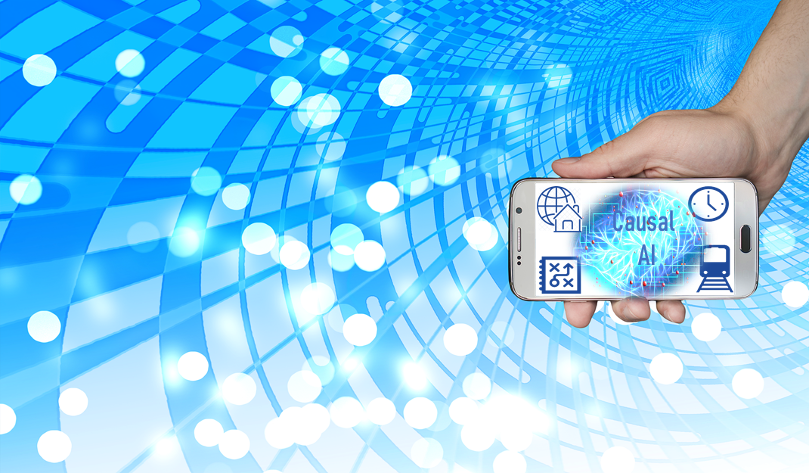
cAIMBER: Causal Artificial Intelligence for Human Mobility Behavior Analysis Using Trajectory Data
Objective
The project envisions a mobile cyber-physical system where people carrying mobile sensors (e.g., smartphones, smartcards) generate large amounts of trajectory data to sense and monitor human interactions with physical and social environments. The project aims to develop a causal artificial intelligence (AI) methodology to analyze and model human mobility behaviour dynamics (decision-making) using individual travel trajectory data and develop the causal diagrams of human mobility behaviour under disturbances that could help design effective strategies for sustainable and resilient urban mobility systems. The research challenges are learning the complex ‘hidden’ human decision-making mechanism from pervasive ‘observed’ trajectories and developing effective, scalable causal AI models and algorithms.
Background
The ever-changing mobility landscape and climate change continue challenging existing operating models and the responsiveness of city planners, policymakers, and regulators. City authorities have growing investment needs that require more focused operations and management strategies that align mobility portfolios to societal goals. The project targets the root cause of traffic (human) and novel analytic techniques to learn and predict human mobility behaviour dynamics from pervasive mobile sensing data that can help cities meet both sustainability challenges (through predicting congestion, emissions, and energy consumption) and improve urban resilience to disruptive events (such as infrastructure failures, natural disasters, or pandemics).
The human mobility area witnessed active developments in two broad but separate fields: transport and computer science. They work with different data, use different methods, and answer different but overlapping questions, i.e., mobility behaviour modelling using ‘small’ data in transport and mobility pattern analysis using ‘big’ data in computer science. A solid bridge between these is beneficial and needed but is still an open challenge. Mobile sensing and information technology have enabled us to collect much mobility trajectory data from human decision-makers. The predictive AI techniques show the potential to learn and predict human mobility using these trajectory data efficiently. However, they continually run up against the limits of what they observe (correlations, not causal relationships), thus hindering any serious applicability for preparedness and response policies for cities without understanding the causal mobility dynamics.
cAIMBER will bridge the two human mobility research streams in Transport Science and Computer Science. Also, it will develop the causal AI methodology, merging the RL and Causal Inference research fields. Integrating interdisciplinary expertise and techniques will derive generalizable insights about human behaviour dynamics that contribute to the scientific communities’ theoretical conceptualization of travel choices and decision-making mechanisms. Practically, cAIMBER conducts extensive empirical analysis using a comprehensive dataset covering different types of system disturbances for seven years. The accumulated knowledge of human mobility under these situational contexts would help city planners and service operators to make more informed decisions for sustainable and resilient travel.
Crossdisciplinary collaboration
The researchers in the team represent the KTH School of Architecture and Built Environment (ABE), Civil and Architectural Engineering Department, Transport Planning Division and KTH School of Engineering Science (SCI), Mathematics Department, Mathematics for Data and AI Division. Strategic research partners at KTH iMobility Lab and MIT Transit Lab support the project.
Watch the recorded presentation at the Digitalize in Stockholm 2023 event:
Contacts
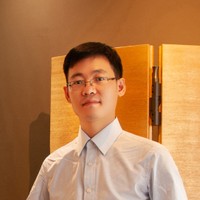
Zhenliang Ma
Assistant Professor in Traffic Engineering, Division of Transport Planning at KTH, ABE School, PI of project DIRAC: DynamIc uRban roAd traffiC noise simulation model using passive and publicly available data, Co-PI of project cAIMBER: Causal Artificial Intelligence for Human Mobility Behavior Analysis Using Trajectory Data, Digital Futures Faculty
+46 8 790 48 14zhema@kth.se
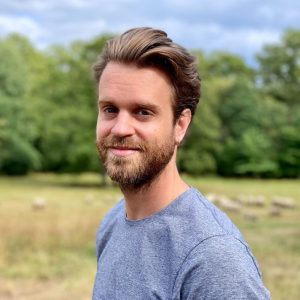
Liam Solus
Assistant Professor at KTH SCI, Working group Learn, Co-PI of project cAIMBER: Causal Artificial Intelligence for Human Mobility Behavior Analysis Using Trajectory Data, Digital Futures Faculty
+46 8 790 65 85solus@kth.se