Fast Distributed Learning based on Adaptive Gradient Coding with Convergence Guarantees
January 2024 – December 2025
Objective
This project aims to propose innovative distributed learning methods based on adaptive gradient coding techniques. Within this framework, workers’ participation is fluidly adjusted in real-time during training to enhance learning performance under practical constraints. We will offer rigorous theoretical proofs to ensure the convergence of the proposed methods, solidifying their reliability. We will also test the performance of the proposed methods on both simulated and actual datasets in real-world scenarios. This evaluation will benchmark the effectiveness of our techniques and underscore their superiority over current practices.
Background
In the framework of distributed learning, a central server aggregates computational results from various workers to update the trained model. However, in practical scenarios, “stragglers”—workers who are slow or unresponsive—can significantly impede overall training time. Addressing these slowdowns is crucial for real-time processing requirements in the healthcare and smart transportation sectors. While current distributed learning methods employ gradient coding to mitigate the effects of stragglers, they rely on a fixed number of the fastest workers throughout the entire training process, which have limited flexibility in balancing training time and loss. Based on that, our research question is how to transcend the limitations inherent in existing distributed learning methods and to reduce the training time required to achieve a specified training loss.
About the Digital Futures Postdoc Fellow
Chengxi Li received a PhD in 2022 from the Department of Electronic Engineering at Tsinghua University and a bachelor’s degree in 2018 from the University of Electronic Science and Technology of China. Her research interests lie in distributed learning, federated learning, signal processing and information theory.
Main supervisor
Mikael Skoglund, Professor, Head of Department, Division of Information Science and Engineering, EECS, KTH.
Co-supervisor
Ming Xiao, Professor, Division of Information Science and Engineering, EECS, KTH.
Contacts
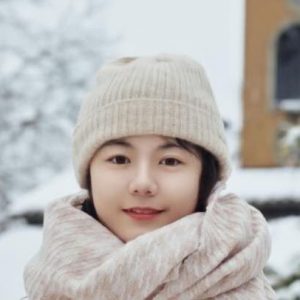
Chengxi Li
Digital Futures Postdoctoral Fellow: Fast Distributed Learning based on Adaptive Gradient Coding with Convergence Guarantees
chengxli@kth.se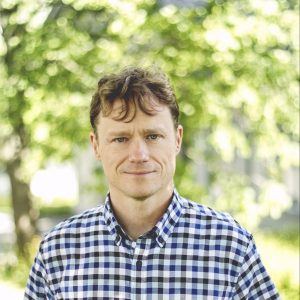
Mikael Skoglund
Professor and Head of Department, Division of Information Science and Engineering at KTH, Member of the Executive Committee, Associate Director Fellows, ICT TNG Director, Working group Cooperate, Co-PI: PERCy, Co-PI: Humanizing the Sustainable Smart City (HiSS), Main supervisor: Fast Distributed Learning based on Adaptive Gradient Coding with Convergence Guarantees, Digital Futures Faculty
+46 8 790 84 30skoglund@kth.se

Ming Xiao
Associate Professor, Division of ISE at KTH EECS, Working group Learn, Co-supervisor: SMART – Smart Predictive Maintenance for the Pharmaceutical Industry, Co-supervisor: Fast Distributed Learning based on Adaptive Gradient Coding with Convergence Guarantees, Former Main supervisor: Intelligent wireless communications and high-accuracy positioning systems, Digital Futures Faculty
+46 8 790 65 77mingx@kth.se