Rapid space-based Detection, Dimensions, and Drivers of forest fires within the context of forest-based Climate solutions (3DFire)
June 2023 – May 2025
Objective
This research (3Dfire) aims to enhance forest fire detection and characterization through advanced remote sensing and AI techniques, facilitating improved management and mitigation strategies within forest ecoregions. Our AI algorithms will streamline the processing of extensive freely available remote sensing data, like Sentinel-1 and -2, to drastically reduce the time and costs associated with fire monitoring and prediction. Consequently, it will provide a comprehensive understanding of fire dynamics within the region. Additionally, “3Dfire” will identify and analyze the risk factors and drivers of fires in the Miombo woodlands, including the impact of extreme climate events and anthropogenic factors on fire severity, frequency, and duration. The project’s outcomes will offer valuable insights to researchers and policymakers responsible for forest fire prevention, minimizing societal and ecological impacts, and promoting sustainable forest management practices. Ultimately, “3DFire” is pivotal in establishing a comprehensive global-scale perspective on AI for forest applications and transitioning towards climate-smart forest management.
Background
Miombo woodlands cover 270 million ha across southern Africa and are increasingly threatened by natural and anthropogenic forces. Despite their importance for biodiversity and 100 million forest-dependent people, this ecoregion receives little attention from the scientific community. The annual forest loss in just a part of the Miombo ecoregion was recently estimated to be more than half a million hectares. Fires are a primary cause of vegetation loss in Miombo, occurring approximately 50% more frequently than in other global ecoregions. They also trigger massive CO2 emissions in sub-Saharan Africa and threaten many ongoing sustainable forest management projects, such as Reducing Emissions from Deforestation and Degradation (REDD). The lack of accurate fire records challenges the estimation of CO2 emissions and evaluation of fire management activities in forests.
Although some dimensions of Miombo’s wildfires, such as frequency and severity, have been explored through coarse- and medium-resolution satellite time-series data from MODIS and Landsat, knowledge of the exact spatial extent, duration, and timing of the fires and their relations with effective driving forces is still lacking. However, fire regimes in miombo are dominated by small burn patches, particularly in the drier regions. Their limited spatial resolution makes it difficult to detect and characterize these small fires using the aforementioned satellites. Extreme climate events significantly elevate the probability of forest fires, while anthropogenic drivers affect all dimensions of fires. Meanwhile, extreme climate events and human pressures on forests are expected to increase as global temperatures rise and the population of southern Africa doubles by 2050. To address these limitations, this research will focus on characterizing and mapping various dimensions of fires, including the severity, frequency, timing, and duration of forest fires in miombo. We will leverage the time series data of fires derived from the Sentinel data and employ deep learning-based algorithms, particularly our developed residual attention UNet5 (RAUNet5).
About the Digital Futures Postdoc Fellow
Zeinab Shirvani is a postdoctoral researcher affiliated with the Division of Geoinformatics at KTH. She obtained a PhD in Remote Sensing/Cartography focusing on forest disturbances from TUD, Germany, in 2020. Before her current position, Zeinab was a postdoctoral researcher at the Swedish University of Agricultural Sciences (SLU) for two years. Zeinab has made significant contributions to remote sensing and machine learning throughout her research career, particularly in mapping woodland fires in tropical dry forests using RAUNet. Her expertise lies in applying geospatial artificial intelligence and remote sensing techniques to study forest disturbances.
Main supervisor
Yifang Ban, Professor and Director of the Division of Geoinformatics at the Department of Urban Planning and Environment at KTH
Co-supervisor
Ulla Mörtberg, Professor and associate professor (docent), Department of Sustainable Development, Environmental Science and Engineering (SEED) at KTH
Watch the recorded presentation at the Digitalize in Stockholm 2023 event:
Contacts
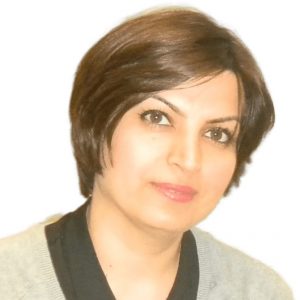
Zeinab Shirvani
Digital Futures Postdoctoral Fellow, Postdoc project: Rapid space-based Detection, Dimensions, and Drivers of forest fires within the context of forest-based Climate solutions (3DFire)
zeinabsh@kth.se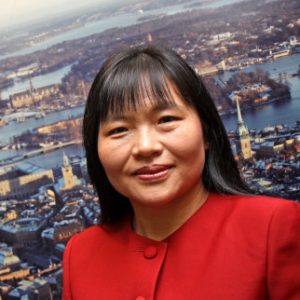
Yifang Ban
Professor and Head of Division Geoinformatics at KTH, Member of the Executive Committee, Associate Director for Dissemination & Impact, PI: EO-AI4GlobalChange, Former Main supervisor: Unraveling the potential of AI and Earth Observation for accurate population predictions in urban regions (POPAI), Former Main supervisor: Fusion of Radar and Optical Remote Sensing Time Series for Wildfire Monitoring with Deep Learning, Digital Futures Faculty
+46 8 790 86 48yifang@kth.se
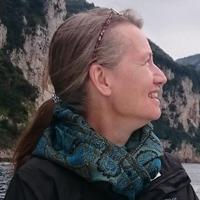
Ulla Mörtberg
Professor and Docent, Sustainable Development, Environmental Science and and Engineering at KTH, Co-PI: EO-AI4GlobalChange, Co-PI: Embedding AI in an innovative geospatial tool to support policy for clean cooking adoption in low- and middle-income countries, Digital Futures Faculty
+46 8 790 86 08mortberg@kth.se