Extraction of Parkinson’s disease related temporal feature of brain activity
May 2024 – May 2026
Objective
This research aims to extract Parkinson’s disease (PD) related temporal features from brain activity and develop brain activity-based biomarkers for diagnosis and prognosis by leveraging advances in machine learning that can form the basis for classifying PD patients.
Background
PD is the second most common neurodegenerative disorder with debilitating consequences. PD diagnosis is based on behavioural symptoms and chemical/genetic markers. Such a diagnosis can be erroneous and often does not capture the disease severity. Despite being a brain disease, the brain’s electrical activity (e.g. fMRI, EEG/MEG) is completely ignored in PD diagnosis. This oversight is intriguing, considering experimental evidence indicating that PD-related changes profoundly impact the temporal dynamics of brain activity.
While biomarkers derived from brain activity usually focus on frequency domain parameters like oscillations and coherence, temporal features have been surprisingly neglected. In fact, except for certain forms of epilepsy, usually, brain activity is not considered in the diagnosis of brain diseases. However, currently, brain activity-based biomarkers are not specific enough. This may be because these are defined in the frequency domain (e.g. oscillations and coherence). Unlike in epilepsy diagnosis, temporal features of brain activity are ignored. Therefore, in my project I would like to change this. I hypothesize that brain region-specific temporal features will provide highly specific information about the disease severity and can provide a more precise diagnosis and prognosis of PD.
About the Digital Futures Postdoc Fellow
Satarupa Chakrabarti, after completing her postgraduation in Computer Science engineering, undertook a PhD degree in Computer Science from KIIT Deemed to be University, Bhubaneswar, India, where she defended her thesis related to designing a generalized epileptic seizure detection method with different feature-extracting techniques from biosensor data that showed the presence of temporal changes. She simultaneously worked as a Junior Research Fellow (JRF) with DST-SERB, India, in an interdisciplinary project (2018–2020).
She has a strong and diverse background in research, with primary research interests in biomedical engineering, signal processing, image processing, space physics, machine learning and deep learning. Satarupa is a Digital Futures Postdoctoral Research Fellow at KTH, based in the Computational Brain Science group at the Division of Computational Science and Technology. Her research experiences involved collaboration with multidisciplinary teams and conducting research on various electrical engineering and space physics projects that led to innovative, beneficial, and cutting-edge endeavours.
Main supervisor
Arvind Kumar, Associate Professor, Division of Computational Science and Technology at KTH
Co-supervisor
Saikat Chatterjee, Associate Professor, Division of Information Science and Engineering at KTH
Contacts

Satarupa Chakrabarti
Digital Futures Postdoctoral Fellow, Postdoc project: Extraction of Parkinson’s disease related temporal feature of brain activity
chakrabartisatarupa@gmail.com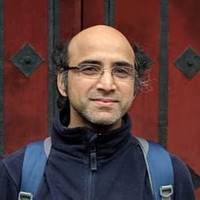
Arvind Kumar
Associate Professor, Division of Computational Science and Technology at KTH, Working group Rich and Healthy Life, Co-PI of research project Digitizing Brain Data for Health and Disease, Digital Futures Faculty
+46 8 790 62 24arvkumar@kth.se
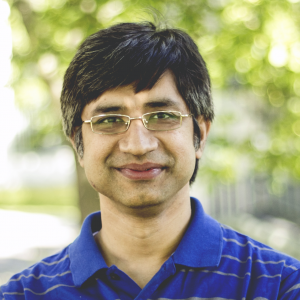
Saikat Chatterjee
Associate Professor, Division of Information Science and Engineering at KTH, Main supervisor: Explainable Machine Learning for Early Warning Systems, Co-PI: Data-Limited Learning of Complex Dynamical Systems, Digital Futures Faculty
+46 8 790 84 78sach@kth.se