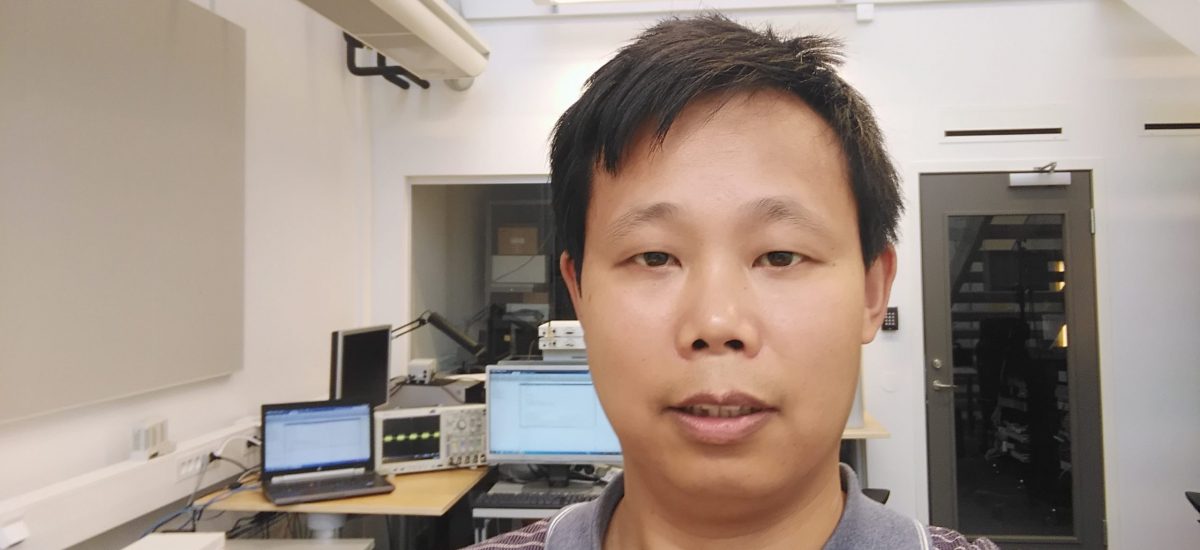
Guessing decoding for CRC is a new research field and has potential to be applied in future smart factory, says Ming Zhan
Ming Zhan – a Member of IEEE – received his master’s degree in communication and information systems from Southwest Jiaotong University, Chengdu, China, in 2004 and a PhD degree from the National Key Laboratory of Science and Technology on Communication, University of Electronic Science and Technology of China, Chengdu, in 2013. From 2016 to 2017, he worked as a Visiting Scholar with the Royal Institute of Technology, Stockholm, Sweden, and ABB Corporate Research Center, Västerås, Sweden.
He is a full professor at Southwest University, China, with a strong background in physical layer design, coding/decoding theories, implementations, and their applications in ultra-reliable and low-latency wireless communications (URLLC). He is recognized for his significant contribution to the WirelessHP (high performance) technology with good academic and industry impacts. Most recently, Prof. Zhan has achieved new progress on the GRAND-MO (guessing random additive noise decoding – Markov order), a novel, promising approach towards URLLC. Zhan is a Digital Futures Scholar-in-residence from August 2023 to March 2024, hosted by Ragnar Thobaben, Associate Professor at KTH and a member of the Digital Futures Faculty.
Hi Ming, you have visited Digital Futures as a Scholar-in-residence in the last couple of months. What made you take on this opportunity?
– We wanted to push forward the research on ultrahigh reliability and low-latency communications from a long period of collaboration with my hosts, Ragnar Thobaben and Zhibo Pang. As we use the guessing decoding strategy to address this challenging topic, complicated testing and frequent discussion are necessary. Ragnar and Zhibo informed me that the Digital Futures project can offer us a good opportunity for closer collaboration. For this purpose, I applied to be a Scholar-in-residence.
You’re interested in the guessing decoding for ultrahigh reliability communication. Why do you find this so fascinating?
– The guessing random additive noise decoding (GRAND) algorithm is a recently proposed strategy for high-reliability short packet transmission. Their decoding algorithms are usually based on their specific coding structures for conventional linear block codes.. Unfortunately, if the channel noise effects are similar to the coding structures, the reliability performance may be degraded. GRAND is a noise-centric decoding algorithm that guesses the channel noise. It is suitable for any linear block codes and can provide optimal error correction capability for short packet transmission.
The cyclic redundancy check (CRC) codes are widely applied in modern communications because of their powerful error detection capability. However, the error correction capability of CRCs is not fully exploited. When Ragnar Thobaben and Zhibo Pang realized the great benefits of CRC decoding by using GRAND, we found this topic very interesting for high-performance control in industrial automation. For short packet transmission in a natural industrial environment, CRCs are used to guarantee the integrity and correctness of the packets. Affected by the strong impulse noise, many packets should be retransmitted if errors are detected. If the errors in CRC-coded packets can be corrected, both the reliability and the transmission efficiency can be improved. To conclude, guessing decoding for CRC is a new research field and has the potential to be applied in future smart factories, which incited us to make a closer collaboration.
What have you encountered in your research on guessing decoding of CRC?
– In our research, we have met a number of difficulties. I would like to summarize these into two issues.
Firstly – how to generate the noise error patterns more efficiently. To guess the noise effects, a long period of testing should be conducted. When the mass data is achieved, it should be processed using smart algorithms. To guess the noise effects for decoding, the noise error patterns are generated from the most likely to the least likely. When the parameters of a specific noise effects family are input, the critical issue is generating the noise error patterns as quickly as possible. In general, the number of noise error patterns is huge. To overcome this problem, we have proposed a high parallel noise-error patterns generation architecture. We are still facing some dilemmas. For example, the power dissipation of the designed high parallel architecture is high, and this difficulty should be optimized in future work.
Secondly – how to evaluate the residual error probability (REP) for CRC using the GRAND algorithm. In essence, the GRAND strategy is to guess the noise effects many times. The packet can pass the CRC error detection if a supposed result is correct. However, this does not mean a packet is valid if it has passed the CRC error detection. If the sum of the channel noise effect and the generated noise error pattern is a legal CRC codeword, the packet can pass the CRC error detection, but the result is wrong. The IEC 61784-3 international standard stipulates that the REP should be no more than 10-10 per hour for safety. When the packet is decoded in a guessed way, the REP is related to the noise error effects, the generated noise error patterns and the adopted CRC-generating polynomial. Such a process is much more complicated than the pure error detection mechanism. Currently, we are deriving the upper bound of REP theoretically. As a primary result, we found the REP worsens as the guessing decoding complexity increases. We hope to get a satisfactory answer.
Finally, how was your summer holiday?
– Summer in Stockholm and Västerås was lovely. Compared to the summertime in Chongqing, China, where the temperature was usually about 40 degrees, this summertime was great. The cool weather, clear water, blue sky, and, most importantly, the friendly colleagues have impressed me greatly. Now, when it is winter time, the duration of daytime is short. I felt a bit uncomfortable in the beginning. But now I have adjusted. Hopefully, I have the chance to enjoy another lovely summer holiday again.
Your scholarship ends on 31 March 2024 – what has your experience been like so far, and what will you do next?
– Digital Futures provided me with an excellent opportunity for collaborative research overseas, enriched my experience for high-quality work, and has built a solid foundation for the next step. We have submitted three manuscripts and applied for one patient. Although this scholarship will soon end, it opened up for future research work, which includes three topics: 1) A highly efficient noise-error patterns generation scheme; 2) Complexity reduced GRAND technique for high throughput processing; and 3) Time-varying adaptive GRAND algorithm for CRC error correction in a natural industrial environment. These works are our focus in 2024.