About the project
Objective
By delivering reliable, local and nearly real-time data about wildlife, the data gathered by FLOX Robotics drones provide insights for data-based wildlife-related decisions to veterinary institutes, nature conservationists, hunting associations, insurance companies and many others. Through AI-assisted identification of wildlife species, the stakeholders have the possibility to track the animal species which are injured, bearing diseases or have been involved in an incident.
The project demonstrates an integrated solution for automated mapping, identification, tracking and, when required, repelling wild animals using autonomous drones with AI-assisted computer vision and ultrasound repellent technology combined with a geographic information system (GIS)-like for data visualization, analysis and decision making.
Background
The problem of wildlife damage is widespread all over the world, from Sweden to Italy, in the US, India and many other countries. Historically, there has been limited means for quantifying the wild animal population, their moving patterns, and the damages they cause. Damage by wild animals to cultivated fields is a major cause of profit loss for farmers in Europe. In Sweden, in 2020, damage caused by wildlife occurred on 17% of the cultivated area for cereals and nearly 28% for starch potatoes. Temporary grasses are Sweden’s largest crop in acreage, and 17% of the cultivated area had some form of wildlife damage in 2020 [www.scb.se]. In Sweden, around 50% of agricultural companies reported damage from the wildstock in 2020, and more than one-third of farmers stated that the wildstock affects their choice of crops.
Wildlife-related damages are widely present not only in agriculture but also in forest areas. The “rooting” and “wallowing” by wild boars also has an environmental impact, destroying vegetation and degrading water quality. For wildlife-related insurance cases, there is often no physical evidence of species involved in the damage. The verification requires too high a burden of proof to be met to receive payments.
The project demonstrates an integrated solution for mapping and, when required, repelling wild animals using autonomous drones with AI-assisted computer vision and ultrasound repellent technology combined with a geographic information system-like (GIS) for data visualization, analysis and decision making. The project solution will help public authorities and decision making bodies to access site-specific identification of wildlife in larger areas, aggregated from separate fields to regional, national and even international levels.
Crossdisciplinary collaboration
The researchers in the team represent RISE Digital Systems and the Department of Computer and System Sciences at Stockholm University.
Watch the recorded presentation at the Digitalize in Stockholm 2023 event:
About the project
Objective
In the DataLEASH project, practically, we develop and test machine learning models, among other methods, to ensure the use of data without the risk of revealing people’s identities or allowing unwanted inferences about them. In a more theoretical approach, we aim at provable guarantees for privacy and take a holistic approach to the legal implications. This implies a quest for finding relevant rules and regulations and illuminating interpretation and application.
The project consortium from KTH, SU, and RISE has a unique set-up in terms of an interdisciplinary and multidisciplinary profile among the researchers, combining perspectives from information theory, legal informatics, language processing, machine learning, cryptography, and systems security.
Background
Digitalization has resulted in more and more data being generated and collected from various sources (such as health care, customer service, surveillance cameras, etc.). The data is valuable for processing and additional analysis to improve predictions and planning. Advances in machine learning have improved this kind of data analysis, while data-protection regulation such as the GDPR has introduced constraints, limiting what data can be used and for what purpose. There is, thus a tension between the utility of data and the privacy of the individuals the data is about.
Cross-disciplinary collaboration
DataLEASH brings together researchers from the School of Electrical Engineering and Computer Science (EECS, KTH), the Department of Computer and Systems Sciences (DSV) and the Department of Law both at Stockholm University and from the Decisions, Network, and Analytics lab at RISE.
Watch the recorded presentation at Digitalize in Stockholm 2022 event:
Activities & Results
Activities, awards, and other outputs
- Speakers at workshops on “AI inom medicinteknik,” session “Vad minns en högparametriserad modell? Organized by Läkemedelsverket, April 6, online with more than 150 participants from industry and regulatory bodies.
- “Tillgängliggörande av hälsodata,” Dec 2021 online with more than 50 participants from four regions participating
- “Digital innovation i samverkan stad, region och akademi,” Oct 2021 online with about 20 participants from KTH, Region and City of Stockholm, plus some KTH internal events.
- Organisation and participation of panel at Nordic Privacy Forum 2022 panel discussing calculated privacy and the interplay between law and tech.
- DataLEASH organizes regular seminars every two months for three years with the City of Stockholm and Region Stockholm about requirements from the stakeholders and the results from our research project.
- SAIS 2022, Swedish AI Society workshop, is organised and paper [BFLSSR22] is presented in this workshop.
- Award: Rise solution for Encrypted Health AI was announced the winner of the Vinnova Vinter competition in the infrastructure category.
Results
Research objectives of DataLEASH are: (i) develop and study privacy measures suitable for privacy risk assessment and utility optimization; (ii) characterization of fundamental bounds on data disclosure mechanisms; (iii) design and study of efficient data disclosure mechanisms with privacy guarantees; (iv) demonstration and testing of algorithms using real-data repositories; (v) study of the cross-disciplinary privacy aspects between law and information technology.
Research achievements and main results of DataLEASH:
- Pointwise Maximal Leakage (PML) has been proposed as a new privacy measure framework. PML has an operational meaning and is robust. Using the framework, several other privacy measures have been derived and their properties have been characterized as well as the relation to existing privacy measures have been established.
- The privacy-preserving learning mechanism PATE has been studied using conditional maximal leakage explaining the cost of privacy. PATE approach has been extended to deal with high-dimensional targets such as in segmentation tasks of MRI brain scans.
- Fundamental bounds on data disclosure mechanisms have been derived considering various pointwise privacy measures. Furthermore, approximate solutions to optimal data disclosure mechanisms have been derived using concepts from Euclidian Information Theory.
- In a cross-disciplinary study between law and tech, we propose and discuss how to relate the legal data protection principles of data minimization to the mathematical concept of a sufficient statistic to be able to deal from a regulatory perspective with the rapid advancements in machine learning.
- Health Bank, a large health data repository of 2 million patient record texts in Swedish has been de-identified. A deep learning BERT model, SweDeClin-BERT, has been created and obtained permission from the Swedish Ethical Review Authority to be shared among academic users. The model SweDeClin-BERT has been used at the University Hospital of Linköping with promising results. Handling sensitive health-related data is often challenging. Proposed Fully Homomorphic Encryption (FHE) to encrypt diabetes data. The proposed approach won the pilot Winter competition 2021–22 organized by Vinnova.
- We created a systematization of knowledge on ambient assisted living (combining the challenges of mobile and smart-home monitoring for health) from a privacy perspective to map out potential issues and intervention points.
- Using a cryptographic approach, we developed distance-bounding attribute-based credentials, which provide anonymity for location-based services, provably resisting attacks.
- We investigated the uses and limitations of synthetic data as a privacy-preservation mechanism. For image data, we developed a framework of clustering and synthesizing facial images for privacy-preserving data analysis with privacy guarantees from k-anonymity and found trade-off choice points with analysis utility. In a different work on facial images, we proposed a novel approach for the privacy preservation of attributes using adversarial representation learning. This work removes the sensitive facial expressions and replaces them with an independent random expression while preserving facial features. For tabular data, we investigated across several datasets whether different methods of generating fully synthetic data vary in their utility a priori (when the specific analyses to be performed on the data are not known yet), how closely their results conform to analyses on original data a posteriori, and whether these two effects are correlated. We found classification tasks when using synthetic data for training machine-learning models more promising in terms of consistent accuracy than statistical analysis.
In the interplay between information technology and law, the project itself has been a testbed, given the personal data processing in research of this kind. Quite often, there is a challenge merely to find the governing legal framework. Practical experiences and theoretical studies can be a sign of this. However, much research today is concentrated on specific data protection regulations. The reasoning above boils down to a broadened approach to GDPR.
Publications
We like to inspire and share interesting knowledge…
- Vakili, T., Hullmann T., Henriksson A. and H. Dalianis. 2024. When Is a Name Sensitive? Eponyms in Clinical Text and Implications for De-Identification. To be presented at the CALD-pseudo Workshop at the 18th Conference of the European Chapter of the Association for Computational Linguistics, EACL 2024, Malta.
- Ngo, P., Tejedor M., Olsen Svenning T., Chomutare T., Budrionis A. and H. Dalianis. 2024. Deidentifying a Norwegian clinical corpus – An effort to create a privacy-preserving Norwegian large clinical language model. To be presented at the CALD-pseudo Workshop at the 18th Conference of the European Chapter of the Association for Computational Linguistics, EACL 2024, Malta.
- Lamproudis, A., Mora, S., Olsen Svenning T., Torsvik T., Chomutare T., Dinh Ngo P. and H. Dalianis. 2023. De-identifying Norwegian Clinical Text using Resources from Swedish and Danish. Proceedings of AMIA 2023, Annual Symposium, November 11-15. New Orleans, LA, USA, link.
- Vakili, T. and H. Dalianis. 2023. Using Membership Inference Attacks to Evaluate Privacy-Preserving Language Modeling Fails for Pseudonymizing Data. Proceedings of the 24th Nordic Conference on Computational Linguistics (NoDaLiDa 2023). Faroe Islands, May 22-24, 2023, link.
- Vakili, T., Lamproudis, A., Henriksson, A. and H. Dalianis. 2022. Downstream Task Performance of BERT Models Pre-Trained Using Automatically De-Identified Clinical Data. In the Proceedings of the 13th International Conference on Language Resources and Evaluation, LREC 2022, Marseille, France, pp. 4245–4252, link.
- Vakili, T. and H. Dalianis 2022, Utility Preservation of Clinical Text After De-Identification. In the Proceedings of the 21st Workshop on Biomedical Language Processing (pp. 383-388) in conjunction with ACL 2022, Dublin, Ireland, link.
- Sara Saeidian, Giulia Cervia, Tobias J. Oechtering, Mikael Skoglund, Quantifying Membership Privacy via Information Leakage, IEEE Transactions Information Forensics and Security. Vol.16, pp. 3096-3108, 2021, link.
- Sara Saeidian, Giulia Cervia, Tobias J. Oechtering, Mikael Skoglund, Optimal Maximal Leakage-Distortion Tradeoff. Information Theory Workshop (ITW) 2021 IEEE, pp. 1-6, 2021, link.
- Vakili, T. and H. Dalianis. 2021. Are Clinical BERT Models Privacy-Preserving? The Difficulty of Extracting Patient-Condition Associations. In the Proceedings of the Association for the Advancement of Artificial Intelligence AAAI Fall 2021 Symposium in HUman partnership with Medical Artificial iNtelligence (HUMAN.AI), November 4-6, 2021, pdf.
- Lamproudis, A., Henriksson, A. and H. Dalianis. 2021. Developing a Clinical Language Model for Swedish: Continued Pretraining of Generic BERT with In-Domain Data. In the Proceeding of RANLP 21: Recent Advances in Natural Language Processing, 1-3 Sept 2021, Varna, Bulgaria, pdf.
- Grancharova, M. and H. Dalianis. 2021. Applying and Sharing pre-trained BERT-models for Named Entity Recognition and Classification in Swedish Electronic Patient Records. In the Proceedings of the 23rd Nordic Conference on Computational Linguistics, NoDaLiDa 2021, Iceland, May 31 – June 2, 2021, pdf.
- Dalianis, H. and H. Berg. 2021. HB Deid – HB De-identification tool demonstrator. In the Proceedings of the 23rd Nordic Conference on Computational Linguistics, NoDaLiDa 2021, Iceland, May 31 – June 2, 2021, pdf.
- Berg, H., Henriksson, A., Fors, U. and H. Dalianis. 2021. De-identification of Clinical Text for Secondary Use: Research Issues. In the proceedings of HEALTHINF 2021, 14th International Conference on Health Informatics Feb 11-13, 2021, pdf.
- Grancharova, M., Berg, H. and H. Dalianis. 2020. Improving Named Entity Recognition and Classification in Class Imbalanced Swedish Electronic Patient Records through Resampling. Compilation of abstracts in The Eight Swedish Language Technology Conference (SLTC-2020), Göteborg, pdf.
- Berg, H., A.Henriksson and H. Dalianis. 2020. The Impact of De-identification on Downstream Named Entity Recognition in Clinical Text. In Proceedings of the 11th International Workshop on Health Text Mining and Information Analysis, Louhi 2020, in conjunction with EMNLP 2020, (pp. 1-11), pdf.
- Berg, H., Henriksson, A., Fors, U. and H. Dalianis. De-identification of Clinical Text for Secondary Use: Research Issues. Presented at the Healthcare Text Analytics Conference HealTAC 2020, April 23, London.
- Berg, H. and H. Dalianis. 2020. A Semi-supervised Approach for De-identification of Swedish Clinical Text. Proceedings of 12th Conference on Language Resources and Evaluation, LREC 2020, May 13-15, Marseille, pp. 4444‑4450, pdf.
- Berg, H., T. Chomutare and H. Dalianis. 2019. Building a De-identification System for Real Swedish Clinical Text Using Pseudonymised Clinical Text. In the Proceedings of the Tenth International Workshop on Health Text Mining and Information Analysis, Louhi 2019, in conjunction with Conference on Empirical Methods in Natural Language Processing, (EMNLP) November 2019, Hongkong, ACL, pp 118-125, pdf.
- Berg, H. and H. Dalianis. 2019. Augmenting a De-identification System for Swedish Clinical Text Using Open Resources (and Deep learning). In the Proceedings of the Workshop on NLP and Pseudonymisation, in conjunction with the 22nd Nordic Conference on Computational Linguistics (NoDaLiDa), Turku, Finland, September 30, 2019, pdf.
- Dalianis, H. 2019. Pseudonymisation of Swedish Electronic Patient Records Using a Rule-based Approach. In the Proceedings of the Workshop on NLP and Pseudonymisation, in conjunction with the 22nd Nordic Conference on Computational Linguistics (NoDaLiDa), Turku, Finland, September 30, 2019, pdf.
Videos & Presentations
Watch recorded videos and download the presentations…
VIDEO RECORDINGS
Presentation at Digitalize in Stockholm 2022
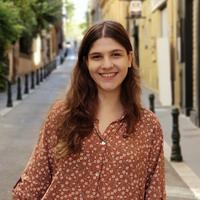
Research: Privacy-preserving data analysis. We apply tools from information theory to problems related to privacy-preserving data analysis
Speaker: Sara Saeidian, PhD student, saeidian@kth.se
Supervisors: Tobias J. Oechtering, Mikael Skoglund
Click here to watch the recorded video presentation on “Privacy-preserving data analysis”
OUR PRESENTATIONS
Quantifying Membership Privacy via Information Leakage
Sara Saeidian, Giulia Cervia, Tobias J. Oechtering, Mikael Skoglund, “Quantifying Membership Privacy via Information Leakage, IEEE Transactions Information Forensics and Security, Vol.16, pp. 3096-3108, 2021.
Machine learning models are known to memorize the unique properties of individual data points in a training set. This memorization capability can be exploited by several types of attacks to infer information about the training data, most notably, membership inference attacks. In this work, we propose an approach based on information leakage for guaranteeing membership privacy. Specifically, we propose to use a conditional form of the notion of maximal leakage to quantify the information leaking about individual data entries in a dataset, i.e., the entrywise information leakage.
We apply our privacy analysis to the Private Aggregation of Teacher Ensembles (PATE) framework for privacy-preserving classification of sensitive data and prove that the entrywise information leakage of its aggregation mechanism is Schur-concave when the injected noise has a log-concave probability density. The Schur-concavity of this leakage implies that increased consensus among teachers in labelling a query reduces its associated privacy cost. We also derive upper bounds on the entrywise information leakage when the aggregation mechanism uses Laplace distributed noise.
DOWNLOAD THE PRESENTATION HERE: Quantifying Membership Privacy via Information Leakage
About the project
Objective
The collaborative project DataLEASH in Action aims to develop novel methods that enable the sharing and learning from data. Legal privacy concerns often prevent implementations of technical solutions so that case studies (sandbox pilots) involving legal and technical competences as proposed in this impact project are seen as the most promising strategy forward. These case studies are pivotal in understanding the nuances of legal requirements and developing technically feasible solutions. The objective is to strike a balance where legal requests are not overly demanding yet necessitate state-of-the-art technical solutions.
Background
Digitalization has resulted in more and more data being generated and collected from various sources (such as health care, customer service, surveillance cameras, etc.). The data is valuable for processing and additional analysis to improve predictions and planning. Advances in machine learning have improved this kind of data analysis, while data-protection regulation such as the GDPR has introduced constraints, limiting what data can be used and for what purpose. There is, thus a tension between the utility of data and the privacy of the individuals the data is about.
Cross-disciplinary collaboration
DataLEASH in Action brings together researchers from the School of Electrical Engineering and Computer Science (EECS, KTH), the Department of Computer and Systems Sciences (DSV) and the Department of Law both at Stockholm University
Objective
With the integration of information and communications technology and intelligent electric devices, substation automation systems (SAS) greatly boost the efficiency of power system monitoring and control. However, substations also bring new vulnerabilities at the frontier of a bulk power system’s wide-area monitoring and control infrastructure. They are known to be attractive targets for attackers. In this project, we will research, develop, and validate algorithms that defend against cyberattacks that aim to disrupt substation operations by maliciously changing measurements and/or spoofing spurious control commands.
We propose multiple use-inspired AI innovations that crucially leverage concurrent capabilities of SAS to transform the cyber security of power systems, including (i) a framework that synergizes optimization-based attack modelling with inverse reinforcement learning for multi-stage attack detection, (ii) a decision-focused distributed CPS modelling approach, and (iii) a mathematical program with equilibrium constraints framework of adversarial unlearning for spoofing detection.
Background
In the IEC 61850-based Substation Automation System (SAS), integrating computing and communication technologies with Intelligent Electric Devices (IEDs) greatly enhances the efficiency of power system monitoring and control. The fast-growing connectivity via wide area networks (WAN) enables powerful automation functions but also brings cyber vulnerabilities concerning new attack vectors. The substations are known to be attractive targets for attackers since they form the frontier of the wide-area monitoring and control infrastructure of a bulk power system, which consists of a Supervisory Control And Data Acquisition (SCADA) system, an Energy Management System (EMS), and a control centre.
Cyberattacks at SASs may be performed by maliciously changing measurements from IEDs and merging units (MUs) and/or spoofing spurious control commands for one or more switching devices from IEDs. An attack can alter a device’s configuration even if commands and data comply with syntax, protocol, and the targeted device. The vulnerabilities of the modern grid are many, as described in a National Academies Report.
Crossdisciplinary collaboration
Anomaly detection can reduce cyber threats to substations and improve root cause analysis. Traditional anomaly data detection heavily relies on human experts to design rule-based detection mechanisms, which can be time-consuming, inefficient, less adaptive, and labour-intensive. More recently, sophisticated anomaly detection methods have been reported in the literature. Still, they largely ignore the special characteristics of attacks on SAS and practical system-level constraints on communication and computation.
Transformative and disruptive applications of use-inspired AI for SAS anomaly detection are in their infancy. The proposed project is among the first known efforts to develop and demonstrate AI-enabled SAS anomaly data detection that crucially leverages the cross-disciplinary collaboration between substation Information engineering and Communications Technology (especially distributed machine learning) for cyber defence.
The project is a collaboration between the University of California Berkeley, Virginia Tech and KTH Royal Institute of Technology.
Watch the recorded presentation at the Digitalize in Stockholm 2023 event:
Objective
We propose a solution using machine learning and test generation, leveraging machine learning expertise from UIUC and testing and verification from KTH. Unlike previous approaches, we focus on explainable AI in our safety cage so that the cage itself and its effects on network traffic can be inspected and validated. Lightweight approaches guarantee that our safety cage can be embedded in programmable networks or operating system kernels. Machine learning will learn behavioural models that have their roots in formal modelling (access policies, protocol states, Petri Nets) and thus are inherently readable by humans. The test-case generation will validate diverse traces against the model and showcase potential malicious behaviour, validating both positive and negative outcomes.
Background
Industrial robots usually operate within a “safety cage” to ensure that a robot does not harm workers. We need the same type of security, simple and explainable, for IT systems. Novel mechanisms that can be embedded in the network, such as through hardware-accelerated programmable networks or kernel extensions, enable this type of security at the network level.
Crossdisciplinary collaboration
The project is a collaboration between the University of Illinois at Urbana-Champaign and the KTH Royal Institute of Technology. KTH will combine its experience in testing and verification with UIUC’s expertise in machine learning.
Watch the recorded presentation at Digitalize in Stockholm 2022 event:
About the project
Objective
The team will address five objectives regarding cyberattacks on power systems based on state-of-the-art AI methods: (1) designing graph neural networks that can process power data to learn the state of the system and detect cyberattacks; (2) developing AI algorithms that utilize image recognition techniques using convolutional neural networks to detect denial of view and image replays resulting from cyberattacks; and (3) developing optimization techniques to robustify previously designed neural networks against adversarial data. Selecting power system operating points and policies through attack-aware methods creates a resilient system. If an attack is not immediately sensed, operating from such a position of strength buys time for detection algorithms. Objectives 4 and 5 aim to develop attack-aware AI methods via distributionally robust optimization and cascading failure analysis.
Background
The operation of power systems is becoming data-centric to improve the efficiency, resiliency, and sustainability of power systems and address climate change. Major operational problems, such as security-constrained optimal power flow, contingency analysis, and transient stability analysis, rely on the knowledge extracted from sensory data. Data manipulation by a malicious actor tampers with grid operation, with catastrophic consequences, including physical equipment damage and cascading failures. Developing frameworks and methodologies that help power operators protect the power grid against such malicious attacks is paramount to national security.
Crossdisciplinary collaboration
The project is a collaboration between the University of California Berkeley, California Institute of Technology, KTH Royal Institute of Technology and Electric Power Research Institute. Assistant Professor Jan Kronqvist leads the research in the Department of Mathematics at KTH. At KTH, the research is focused on developing optimization techniques to robustify previously designed neural networks against adversarial data and the fundamental mathematical theory needed to develop such optimization techniques.
Contacts at other participating institutes:
Javad Lavaei, Associate Professor, Industrial Engineering and Operations Research, University of California, Berkeley
Somayeh Sojoudi, Assistant Professor of Electrical Engineering & Computer Science, University of California, Berkeley
Steven Low, Professor of Computing and Mathematical Sciences and Electrical Engineering, California Institute of Technology
Jeremy Lawrence, Principal Technical Leader at Electric Power Research Institute, Electric Power Research Institute
Watch the recorded presentation at the Digitalize in Stockholm 2023 event:
About the project
Objective
We propose to develop computationally efficient machine learning algorithms and tools for attack detection and identification based on a novel, scalable representation of the physical system state, the communication protocol state and the IT infrastructure’s security state maintained based on noisy observations and measurements from the physical and the IT infrastructure. The key contribution is to learn a succinct representation of the security state of the IT infrastructure that allows computationally efficient belief updates in real-time and enables jointly accounting for the evolution of the state of the physical system, communication protocols, and infrastructure for accurate detection of attacks and identification through causal reasoning based on learnt dependency models.
The research will help address questions such as achieving real-time situational awareness in complex IT infrastructures, developing anomaly detectors with low false positive and false negative rates, and using information about IT infrastructure to improve attack identification. The project leverages the expertise of three research teams from KTH, UIUC, and MIT, with extensive expertise in cyber-physical systems security, smart grids, and anomaly detection.
Background
Modern SCADA systems rely on IP-based communication protocols that are primarily event-driven and follow a publish-subscribe model. The timing and content of protocol messages emerge from interactions between the physical system state and the protocol’s internal state – as an effect, traditional approaches to anomaly detection result in excessive false positives and, ultimately, alarm fatigue.
Crossdisciplinary collaboration
The project is a collaboration between the KTH Royal Institute of Technology, the University of Illinois at Urbana-Champaign and MIT.
Watch the recorded presentation at the Digitalize in Stockholm 2023 event: