About the project
Objective
This research will develop design considerations and policy recommendations for designing with and applying (inter)personal and intimate data. The project will follow a participatory approach centered around people’s experiences interacting with and sharing intimate technologies that collect and store (inter)personal data.
Background
In today’s digital society, most people routinely interact with connected products and services that collect and indefinitely store personal data. Increasingly, these products and services — and the data they produce — permeate intimate spaces, such as smart vibrators collecting sensor data about people’s arousal and orgasm, and AI romance chatbots collecting self-reported information about people’s mental and sexual health. Moreover, these intimate spaces are often shared and relational. For instance, a connected voice assistant in a shared household collects data from the primary user, other household members, and even occasional visitors. Thus, data becomes both intimate and (inter)personal, shaped by and shared across (inter)personal relationships around shared experiences and spaces.
These characteristics raise critical questions for data, design, and policy. Although connected intimate technologies — and the data they produce — are often designed for individual use(rs), their use is often shared and relational: How can we design intimate technologies that empower their users to care for and share their data? Similarly, regulations such as the GDPR established several rights to empower individuals to control their data, such as the right to access: Who should access (inter)personal data?
About the Digital Futures Postdoc Fellow
Alejandra Gómez Ortega is a Design and Human-Computer Interaction researcher. She holds a PhD in Industrial Design Engineering from the Delft University of Technology in The Netherlands. Her research investigates individual experiences interacting with and sharing intimate data, privacy perceptions and considerations around data, and data themselves through playful and creative approaches. Alejandra has applied various methods and approaches in her design research journey, including Participatory Design and Research through Design. Alejandra enjoys designing, developing, deploying, and exhibiting provocative artifacts and digital prototypes that enable individuals and communities to experience a specific situation as a starting point for reflection and discussion.
Main supervisor
Airi Lampinen, Associate Professor, Department of Computer and Systems Sciences (DSV), Stockholm University.
Co-supervisor
Madeline Balaam, Professor, Division of Media Technology and Interaction Designs, KTH.
About the project
Objective
The aim of this project is to analyse the environmental impacts of increased digitalization and the use of Information and Communication Technologies. The project can include both method development and case studies. The impacts will be analysed using life cycle assessment and life cycle thinking. Case studies can vary on different scales and include specific devices, applications and sectoral assessments. Initially, the focus will be on climate impacts and energy use, but it may also be broadened to a larger spectrum of environmental impacts. Assessments will include the direct impacts of ICT but also different types of indirect impacts, including rebound effects.
Background
The ICT sector has an environmental footprint. The future development of this footprint is debated, and it is important that the discussions have a scientific basis. Digitalisation may be a tool for reducing environmental impacts. By improving efficiencies and dematerialising products and services, new ICT applications can reduce the footprints of other sectors. More studies are, however, needed in order to understand when this actually leads to decreased impacts and when there is a risk for indirect rebound effects that increase use and footprints. Environmental life cycle assessment is a standardised method for assessing potential environmental impacts of products, services and functions “from the cradle to the grave”, i.e. from the extraction of raw materials via production and uses to waste management. It is used for analysing the environmental footprints, i.e. the direct impacts, of ICT. It can also be used for analysing different types of indirect effects.
Partner Postdocs
After working in the industry on large-scale refrigeration and heat pump systems and as an entrepreneur with solar pumps, Shoaib Azizi undertook a master’s program in Sustainable Energy Engineering at KTH. He moved to Umeå in northern Sweden for a multi-disciplinary PhD project on energy-efficient renovation of buildings. His PhD included research on the opportunities for digital tools to improve management and energy efficiency in buildings. He defended his thesis “A multi-method Assessment to Support Energy Efficiency Decisions in Existing Residential and Academic Buildings” in September 2021. Now Shoaib is a Digital Futures Postdoc researcher in digitalization and climate impacts at the Department of Sustainable Development, Environmental Science and Engineering (SEED) at KTH. His research involves lifecycle assessment methodology to understand various aspects of digitalization and its impacts on the environment.
Anna Furberg defended her PhD thesis in 2020 at Chalmers University of Technology. Her thesis, titled “Environmental, Resource and Health Assessments of Hard Materials and Material Substitution: The Cases of Cemented Carbide and Polycrystalline Diamond”, involved Life Cycle Assessment (LCA) case studies and method development. After her thesis, she worked at the Norwegian Institute for Sustainability Research, NORSUS, on various LCA projects and, in several cases, as the project leader. In 2022, she was awarded the SETAC Europe Young Scientist Life Cycle Assessment Award, which recognizes exceptional achievements by a young scientist in the field of LCA. Anna has a Digital Futures Postdoc position in digitalization and climate impacts at the Department of Sustainable Development, Environmental Science and Engineering (SEED) at KTH.
Supervisor
Göran Finnveden is a Professor of Environmental Strategic Analysis at the Department of Sustainable Development, Environmental Sciences and Engineering at KTH. He is also the director of the Mistra Sustainable Consumption research program. His research is focused on sustainable consumption and life cycle assessment, and other sustainability assessment tools. The research includes method development and case studies in different areas, including the environmental impacts of ICT.
About the project
Objective
The SENZ-Lab project develops and validates a cost-efficient, real-time, dynamic sparse sensing approach for urban traffic monitoring and environmental footprint assessment in Stockholm’s Environmental Zone Class 3. Using acoustic sensors and AI-driven modelling, it seeks to establish a 2D digital twin of the city’s traffic, enabling real-time monitoring of noise, air pollution, and vehicle-level activity. The goal is to enhance traffic management, reduce emissions, and support sustainable urban mobility.
Background
As cities expand, noise and air pollution pose significant health risks. Traditional monitoring methods struggle with real-world complexity, requiring new solutions. Building on previous research in Stockholm’s Hornsgatan innovation zone, this project integrates IoT, AI, and real-time traffic simulations to improve monitoring accuracy and inform urban policy. The initiative aligns with Stockholm’s environmental goals and KTH’s strategic pillars of sustainability and digitalization.
Crossdisciplinary collaboration
The project brings together experts from multiple fields, including urban sensing, traffic modeling, AI, and GIS-based visualization. The consortium includes two research teams at KTH specialized in Acoustics and Geoinformatics, supported by the City of Stockholm, combining academic research with real-world urban planning needs. By integrating cutting-edge technology with policy-driven insights, the project provides practical solutions for creating quieter, healthier, and more sustainable cities.
About the project
Objective
Mediverse will showcase how clinical and medical researchers can integrate multimodal health data into a unified knowledge graph. Our showcase will give a live example of advanced data exploration, complex medical searches, and predictive analytics across diverse modalities, such as genomic data, clinical studies, and medical imaging. Leveraging Graph Neural Networks (GNNs) and black-box uncertainty estimation, Mediverse aims to also establish trust in AI-driven healthcare insights, accelerating medical discovery while ensuring transparency and reliability, two instrumental needs in the health sector.
Background
Health data is typically fragmented and siloed across various institutions and data formats, making integration and large-scale analysis highly challenging. Despite regulatory efforts, the problem tends to worsen over the years. At the same time, existing approaches to interoperability struggle to capture the full spectrum of clinical relationships across different medical domains. Mediverse addresses these challenges by embracing data diversity. At its core, our method is based on a unified future-proof hierarchical model built using knowledge graph representations that link diverse healthcare datasets, enabling seamless interaction and in-depth exploration. By incorporating state-of-the-art graph representation learning techniques, Mediverse facilitates cross-ontology mapping, providing a powerful tool for clinicians and researchers to uncover previously inaccessible insights.
Crossdisciplinary collaboration
Mediverse is a collaboration between KTH’s Data Systems Lab (EECS) and the Center for Data-Driven Health (CBH). It brings together valuable expertise in health informatics, knowledge graph modeling, machine learning, and data systems engineering. The project is further strengthened by partnerships with Karolinska Institute, Region Stockholm, and the private sector, enabling real-world validation and deployment within Sweden’s healthcare ecosystem.
About the project
Objective
The project aims to develop a self-powered biodegradable pressure sensor with the potential for wireless data transmission that is tested in vitro under conditions that mimic the in vivo environment of physiological blood flow. The pressure sensor is based on the self-powered triboelectric nanogenerator technology and will combine components that enable high performance and on-demand biodegradation. Sensor validation will be enabled by a hybrid mock circulatory loop: an in vitro system that simulate the dynamics of the healthy and pathological patient’s circulatory system. The method will enable to validate sensor-generated pressure signals against reference pressures generated by a digital patient representation.
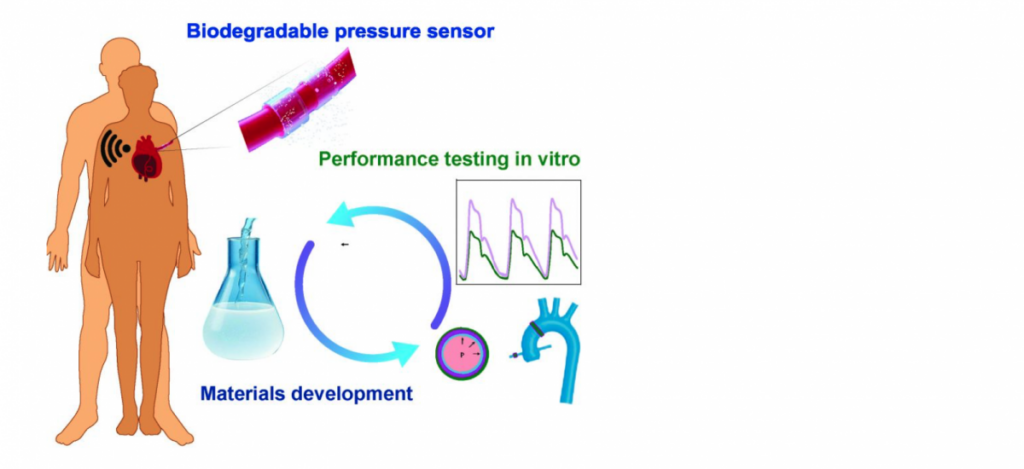
Objective
The project aims to develop a self-powered biodegradable pressure sensor with the potential for wireless data transmission that is tested in vitro under conditions that mimic the in vivo environment of physiological blood flow. The pressure sensor is based on the self-powered triboelectric nanogenerator technology and will combine components that enable high performance and on-demand biodegradation. Sensor validation will be enabled by a hybrid mock circulatory loop: an in vitro system that simulate the dynamics of the healthy and pathological patient’s circulatory system. The method will enable to validate sensor-generated pressure signals against reference pressures generated by a digital patient representation.
- Erica Zeglio, Assist. Prof. in organic bioelectronics / materials chemistry at the Department of Chemistry at SU, is an expert in materials with ionic and electronic conductivity and their application in bioelectronic devices.
- Seraina Dual, Assist. Prof. in Intelligent Health Technologies at the Department of Biomedical Engineering and Health Systems at KTH, is an expert in implantable sensors and robotic systems for prevention and treatment of cardiovascular disease.
About the project
Objective
The project aims to develop analysis and synergy mechanisms for complex systems consisting of high-order interactions, with a particular focus on opinion and societal-scale dynamics on hypernetworks. The ultimate goal is to apply the methodology and insight derived from collective behaviors of social dynamics on hypernetworks and moment-based approaches in societal-scale networked systems to design more efficient and sustainable infrastructures. These include transportation systems, smart grids, and smart buildings, where human decisions and group interactions are pivotal.
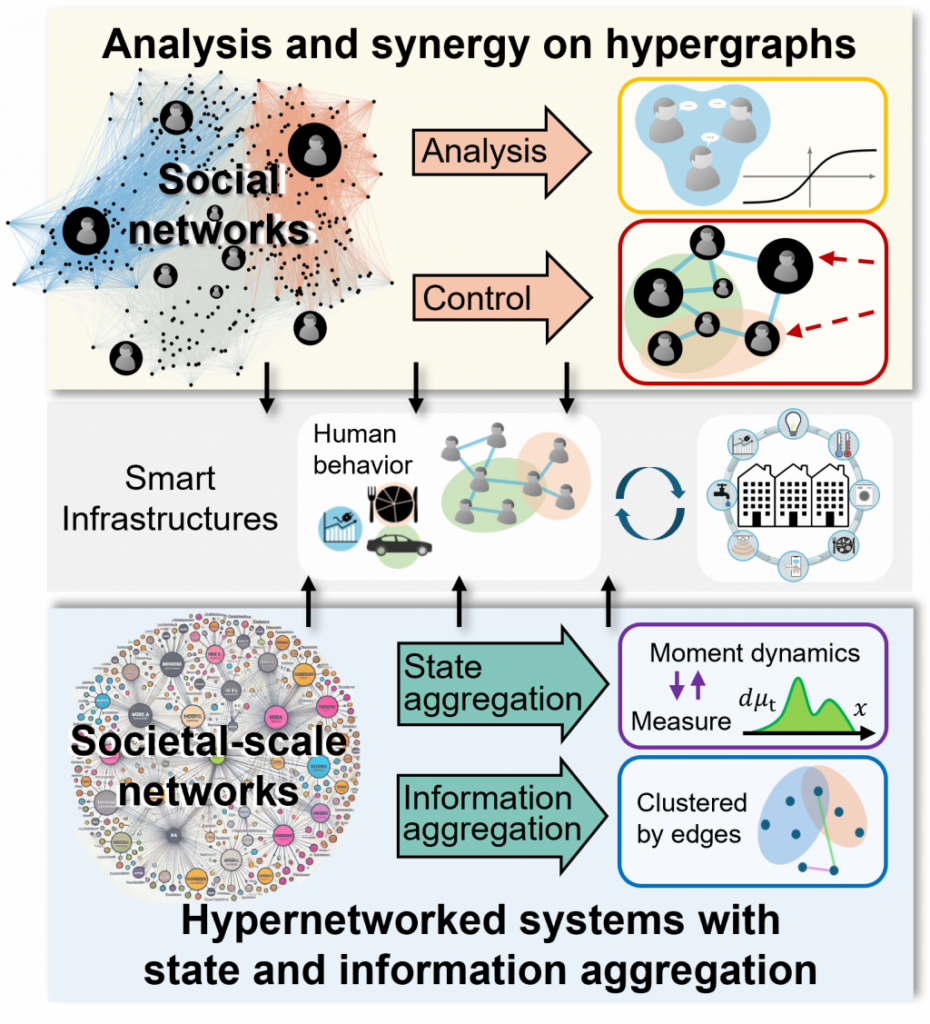
Background
In recent years, there has been a growing interest in studying the collective behavior of systems with higher-order interactions. The motivation stems from a practical need in real-world applications where the phenomena observed in complex systems cannot be adequately captured by considering only pairwise interactions between agents. Instead, these systems require the inclusion of higher-order interactions, often represented by hypergraphs or simplicial complexes. Such a need is evident in numerous applications, ranging from neuron dynamics to protein interaction networks, and from ecological systems to social systems. Understanding how these high-order interactions affect collective behaviors in social dynamics and incorporating their effects into human-involved infrastructures is greatly needed.
Crossdisciplinary collaboration
Our research team is formed by PIs from two KTH Schools, Angela Fontan (KTH/EECS) and Silun Zhang (KTH/SCI). The project team will also include a postdoctoral researcher with a strong background and interest in networked systems, control and systems theory, optimization, and large-scale system modeling.
About the project
Objective
The project envisions a mobile cyber-physical system where people carrying mobile sensors (e.g., smartphones, smartcards) generate large amounts of trajectory data that is used to sense and monitor human interactions with physical and social environments. Built upon the static causal inference results in the cAIMBER project, the CIML4MOB project aims to build causally informed machine learning models for predicting adoption time of individuals and subpopulations and their risks of attrition by input dates. Such dynamic causal models may then drive policy design strategies for lasting behavioral changes (the ultimate purpose of behavior interventions).
Background
The ever-changing mobility landscape and climate change continue to challenge existing operating models and the responsiveness of city planners, policymakers, and regulators. City authorities have growing investment needs that require more focused operations and management strategies that align mobility portfolios to societal goals. The project targets the root cause of traffic (human) and proposes causally informed machine learning to learn and predict human mobility dynamics from pervasive mobile sensing data that helps cities meet both sustainability challenges and improve urban resilience to disruptive events.
The human mobility dynamic problem is defined to predict travel choice decisions given a set of factors, including for example individual traits, travel contexts, and interventions. The research pair project (cAIMBER, 2022-2024) developed the data-driven causal inference method to discover the static causal graph of behavior responses to interventions in public transport. The cAIMBER causal model allows for analysis and prediction of human behavior based on population features, but without regard to when individuals or other subpopulations will adopt the desired behavior of a certain incentivization program. From the perspective of city planning and utility costs, two fundamental questions are (1) how to incentivize early adoption of the desired behavioral shift (adoption time) and (2) given an individual has shifted their behavior, how to prevent reversion to baseline behavior (attrition time). The research consolidator project, CIML4MOB, aims to build upon cAIMBER results to build causally informed machine learning models for predicting adoption time of individuals and subpopulations and their risks of attrition by input dates.
Crossdisciplinary collaboration
The research collaborates between researchers in transportation science and mathematics at KTH.