About the project
Objective
This project aims to develop a Risk Prediction Tool to use as an early warning system in primary care, aimed at cancer using Colorectal Cancer as the first test case. The tool will be based on data collected at primary care units, such as care visits such as history, symptoms, medication, lab tests and results, as well as free text from physician notes. The tool will use machine learning (ML), large language models and advanced statistical analyses to assess the risk of patients having an underlying yet undiagnosed CRC. Despite the promise and potential of primary care data to assess risk and improve screening, very few studies use this potential to better inform diagnostics.
Existing risk assessment tools designed for use in primary care have been developed and validated with a limited dataset (ICD-10 codes that were present in primary care datasets, covering a smaller percentage of patients), but are not yet in clinical use. The project aims to gain an understanding of the most salient data points for assessing risk of CRC in primary care, as well as insight into the potential benefits of such analysis for the healthcare system. Through this analysis, the project also aims to obtain a validated, novel risk prediction tool for CRC, which can be widely deployed and feed into national guidelines for colorectal cancer. By using CRC as the initial test case, the project aims to develop methods and tools through the results of the project which can be modified for other cancers and applications.
Background
A majority of patients with cancer present with early symptoms in primary care, before receiving their diagnosis. Despite the fact that Sweden has fast track for cancer diagnosis since 2015 (cancer care pathway, CCP) primary care physicians lack decision support to assess cancer risk for individual patients. Colorectal cancer (CRC) is the third most common cancer in Sweden. Despite screening, most patients with CRC are diagnosed after symptom presentation. The survival is excellent in patients diagnosed with CRC limited to the bowel wall (stage I-II) and intermediate when it has spread to regional lymph nodes (stage III) while the prognosis is poor in the approx. 25% who are diagnosed with CRC with distant metastases (stage IV).
Cross-disciplinary collaboration
The project is a highly inter-disciplinary collaboration between KTH, Regional Cancer Centrum Stockholm-Gotland, Regional Cancer Centrum Väst and Karolinksa Institutet. The project team comprises primary care clinicians, statisticians, modelers, software engineers and planners at RCC.
About the project
Objective
AsthmaTuner is an existing remote digital tool owned by MediTuner AB in Sweden. AsthmaTuner consists of a handheld spirometer connected to a mobile app that can be used by patients with asthma and other respiratory diseases to diagnose and manage their health condition.
Explainable Artificial Intelligence (XAI) has high potential to enhance existing digital tools previously developed without AI or with black-box AI. The existing AsthmaTuner system does not use any AI so far, and naturally the scope of using black-box AI has a high potential. In this proposed project, we pursue a significant step ahead. We will explore how explainable AI (XAI) will enhance both the diagnostic and management capabilities of the AsthmaTuner system. That means, XAI will help both patient users and their health care providers further improve asthma care, with the target of reduced need for unnecessary health care visits, or costly and potentially deadly asthma attacks or exacerbations. The XAI-based digital tools are expected to prevent further health disparities and will include the addition of social determinants of health to the design, implementation, and evaluation to AsthmaTuner to improve the performance.
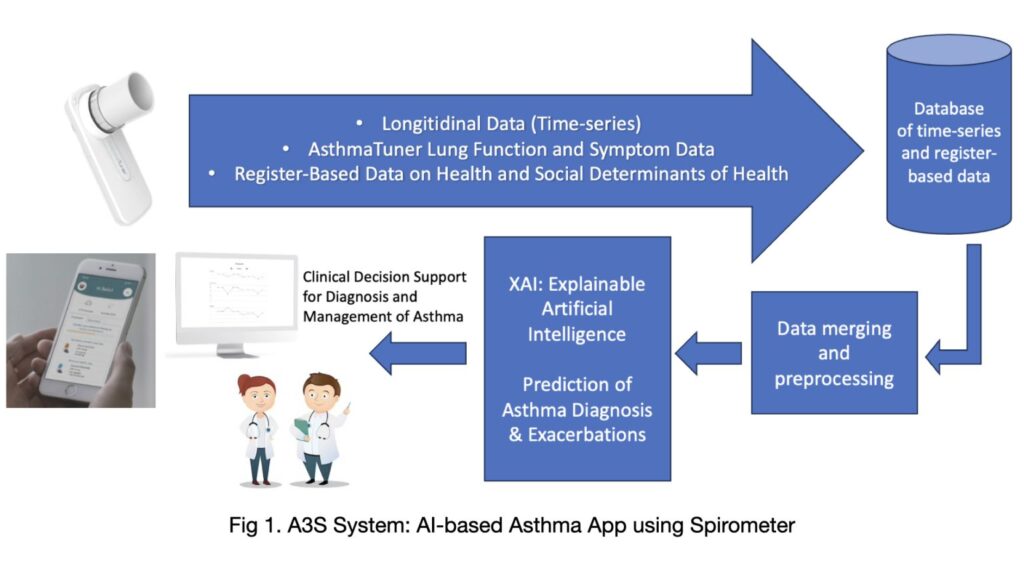
Background
Asthma is a large-scale health care problem, affecting around 10% of the European population, and moreover; asthma and other respiratory diseases are increasing due to environmental deterioration worldwide. Asthma is a complex problem, which is characterized by airway inflammation and respiratory symptoms of wheezing, dyspnea, chest tightness and cough that vary over time and in intensity, together with variable expiratory airflow limitation. Potentially effective ways to address asthma care are to better understand the day-to-day lung function (a longitudinal data) and asthmatic symptoms of individuals and more effectively identify, diagnose, and manage asthma by exploring potential disparities in symptoms, lung function, and outcomes that may exist by social determinants for patients. AsthmaTuner (AT) is the existing, validated digital tool in this regard.
The general purpose of the project is to design an explainable AI (XAI)-based smart AT system that uses a mobile app and a handheld spirometer. We refer to the proposed project as “A3S: AI-based Asthma App using Spirometer”. For the A3S system development, XAI algorithms will perform patient data analysis and guide decisions. The term “explainable” in XAI refers to the ability of these algorithms to provide transparent and interpretable explanations to patients and health care professionals for their predictions or decisions. The XAI algorithms will provide person-centred interventions precluding the onset or minimizing the risk of asthma in disadvantaged groups. It will help to improve respiratory health by training person-centred AI-support in asthma diagnosis and management of asthma in disadvantaged groups based on social determinants. Overall, the A3S project addresses a pressing health care problem with potentially widespread impact.
Cross-disciplinary collaboration
This is a highly collaborative project with a team of data scientists, clinicians, and AI specialists. Partners are PI Magnus Jansson, Co-PIs Saikat Chatterjee, PostDoc Zhendong Wang of EECS KTH, Ioanna Miliou of DSV SU, Docent Björn Nordlund of KUH and KI and his team, along with MediTuner AB.
About the project
Objective
The project aims to develop a system for online rehabilitation of patients with long-term cognitive symptoms after Covid-19.
The project will start with an analysis and concretization of patients’ and clinicians’ demands on the existing pilot system. This information will then be used to develop the system for testing further at the Rehabilitation Medicine University Clinic Stockholm at Danderyd Hospital.
Background
The focus is on patients with severe cognitive problems, e.g. fatigue, concentration problems and memory disorders, after Covid-19. The system to be developed aims to help patients understand more about their symptoms, train to overcome them, and increase their motivation to work with their rehabilitation online.
The project also aims to apply an innovative visualization technology that can support workflows and information flows as well as the integration of applications. The technology can support care personnel so that they can easily receive the results from the patients’ online rehabilitation training and provide support in order to further increase the patients’ motivation.
The project collaborates with the clinical team at Rehabilitation Medicine University Clinic Stockholm, Danderyd Hospital and Visuera Integration.
Crossdisciplinary collaboration
The project partners are Region Stockholm, Danderyd University Hospital, Visuera Integration AB and Telia Company.
Watch the recorded presentation at the Digitalize in Stockholm 2023 event:
About the project
Objective
To develop a mobile application that leverages advanced 3D pose estimation technology for tracking and analyzing a person’s movement outside traditional laboratory settings. This app aims to complement the clinician’s work by enabling remote monitoring and interaction between patients and healthcare providers, thus facilitating data-driven physiotherapy and rehabilitation sessions.
The benefits of such an approach include:
- Adaptive, personalized therapy to each patient’s progress and needs
- Progress tracking highlighting achievements and areas needing attention
- Assisting in correct execution by providing immediate feedback
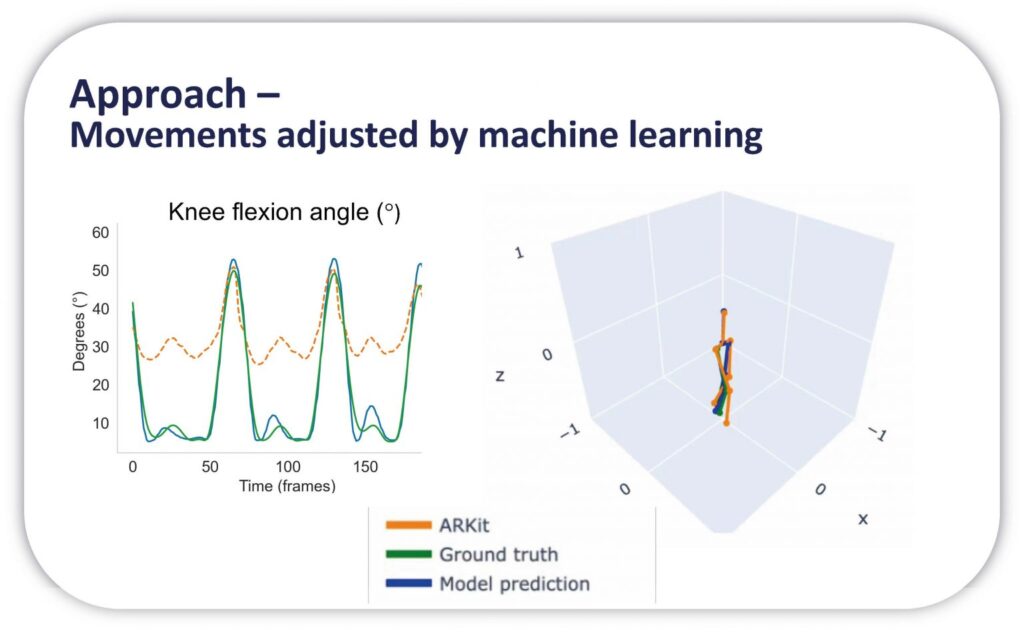
Background
The need for innovative tools that offer quantitative insights into patients’ movements has become increasingly apparent, particularly for remote or home-based physiotherapy and rehabilitation. Traditional methods often rely on in-person assessments that may not fully capture the nuances of a patient’s progress or challenges.
An app that provides accurate, quantitative movement analysis can significantly enhance the clinician’s ability to tailor treatments, monitor progress remotely, and ensure patients perform exercises correctly while reducing the need for frequent in-person visits.
Status
The current app demo utilizes a single smartphone or tablet to capture a person’s 3D movement using the device’s depth camera capabilities. To ensure high accuracy and reliability, the app employs a machine learning model to refine and improve pose estimation based on data collected from a wide range of users. The current implementation primarily targets the lower extremities, focusing on walking. Efforts are underway to broaden the model’s scope to encompass a broader range of movements.
Crossdisciplinary collaboration
The project partners are Innovations Office Region Stockholm and Danderyd University Hospital.
Watch the recorded presentation at the Digitalize in Stockholm 2023 event:
About the project
Objective
The QB-ACT project aims to develop and evaluate an Internet-based psychological intervention integrating Question-Based Learning (QBL) and Acceptance and Commitment Therapy (ACT). It seeks to create an engaging, user-friendly platform for delivering therapy to improve mental health outcomes, reduce dropout rates, and enhance treatment adherence. By conducting a randomized controlled trial, the project will assess its effectiveness in addressing anxiety, depression, and overall well-being. Additionally, it aims to promote accessibility and scalability, providing an innovative alternative to traditional mental health care while supporting broader adoption through stakeholder dissemination and integration into healthcare systems.
Background
The QB-ACT project addresses critical challenges in mental health care: the growing demand for services and insufficient accessibility. Internet-based psychological interventions offer scalable solutions but often face issues like low engagement and high dropout rates. To overcome these barriers, the project integrates the evidence-based framework of Acceptance and Commitment Therapy (ACT) with Question-Based Learning (QBL), a proven methodology from e-learning. ACT fosters mindfulness and value-driven action to improve mental health, while QBL enhances engagement and learning through interactive, problem-solving techniques. By uniting these approaches, QB-ACT seeks to create a transformative digital platform, making therapy more accessible, personalized, and effective.
Crossdisciplinary collaboration
The proposed research project unites a multidisciplinary team with expertise spanning clinical psychology, Internet-based psychological treatments, large-scale e-learning technology, instructional design, online education, and the OLI Torus e-learning platform, ensuring a comprehensive and innovative approach to digital mental health interventions.
About the project
Objective
The project aims to develop a model-based decision-making tool for planning, designing and improving patient flows at the Karolinska University Hospital. The tool will include a prediction model, integrating clinical data, production data, and expert knowledge of the patient flows through the emergency department and the hospital. In addition, a network-based simulation model will be integrated to facilitate capacity and resource planning to improve patient care, decisions on handling and resource utilisation.
Background
Hospital emergency departments play a central role in healthcare systems and patient flow logistics throughout the system. Delivery of emergency care is resource and knowledge-intensive and requires close alignment with all other hospital functions and departments. Emergency healthcare is a complex system with high variability in patient characteristics, disease profile, processes and outcomes. This is a challenge for change work aimed at improving efficiency.
Little or no work has combined clinical and production data into process models to inform healthcare planning. Current models treat the emergency department as an independent leverage point without considering downstream bottlenecks in hospital wards. Overcoming these methodological limitations is important for informing hospital resource planning.
Crossdisciplinary collaboration
The project partners are Karolinska University Hospital, Region Stockholm, and KTH.
Watch the recorded presentation at the Digitalize in Stockholm 2023 event:
About the project
Objective
This Seed project allows three groups – Prevent, Nano and Trauma – to prepare one larger grant application each, preferably to the ERC ( European Research Council) Synergy program.
Background
Prevent: A key to improving global health is to prevent diseases, but even for those with a known high risk, it remains a challenge to intervene before an illness arises. The purpose of our project is the creation of molecular home-sampled digital diaries to enable the prevention of diseases using the case study of Type 1 diabetes. We propose to build these diaries on frequent blood micro-sampling and reveal the changes in the circulating proteome during the pre-symptomatic stage. Applying artificial intelligence (AI) to such data will uncover the mechanisms behind the onset and possible interventions of T1D. While this project is focused on T1D, we expect our results to apply to many more diseases and provide a unique bridge for clinical care at home.
Nano: Detecting and identifying biomolecules such as DNA, RNA, and proteins at the single molecule level have exciting applications for biology, medicine, and data storage. Solid-state nanopores (ssNPs) have emerged as powerful electrical devices for detecting biomolecules at the single-molecule level. However, the realization of solid-state pores with sub-10 nm diameters remains a major obstacle to practical applications. The translocation of biomolecules through solid-state nanopores is too fast to permit reliable molecule sensing. To address these limitations, we aim to push the frontiers of solid-state nanopore sensors for detecting, fingerprinting, and sequencing biomolecules. For scalable manufacturing of nanogaps and pores with controllable diameters ranging from 1-20 nm, we will utilize an original crack-lithography methodology conceived by our teams (Stemme and Herland) [1, 2]. We will explore dynamic protein interactions of different existing affinity pairs (Hober) using our pores. Furthermore, we will develop novel model-based machine learning methods to classify biomolecules from the noisy nanopore sensor signals (Jaldén).
Trauma: Abusive head trauma (AHT) (also called Shaken Baby Syndrome) is a leading cause of fatality in infants who experience a non-accidental injury. The financial cost of AHT is substantial, not to mention the tragedies for the surviving infants facing life-long disabilities. Its diagnosis is often subjective and highly controversial. It has challenged clinicians and forensic practitioners during the past 60 years, often arousing heated debates that are ongoing today, including an intensive debate in Sweden. This project aims to bring multidisciplinary expertise to develop innovative tools toward an objective diagnosis of AHT by integrating computational biomechanics, biomedical sensors, advanced imaging, and legal aspects.
Another important focus of this project naturally connected to the work on AHT is to develop two innovative devices: (1) a new approach to prevent pre-term neonate intracranial ventricular haemorrhage (IVH); (2) a new protective headband for babies at the learn-to-walk stage.
Crossdisciplinary collaboration
The researchers in the team represent the KTH Royal Institute of Technology and Stockholm University from Digital Futures Working Group Rich and Healthy Life.