About the project
Objective
In this project, we propose to extend current assessments of the various synergies and trade-offs among the Sustainable Development Goals (SDGs) proposed by the United Nations (UN) via methods based on artificial intelligence (AI). The project will also strengthen the collaboration between Digital Futures and the KTH Climate Action Centre.
The proposed research project is relevant due to the pressing need to develop a policy that enables, rather than inhibits, sustainable development. Furthermore, including AI-based methods in governance actions and development programs is essential.
Background
This is currently an active area of research, where several studies have started to document the interactions among SDGs systematically. As this type of work is extremely relevant for policymakers, as well as to guide promising research directions to explore by funding agencies, we will:
- Compare AI-based analyses with expert-based studies.
- Systematically assess (via AI methods) synergy and trade-offs connected to climate policy decisions in the context of the SDGs.
- In the final stage, employ reinforcement-learning-based methods to design novel and efficient strategies for policy development, maximizing the opportunities among SDG actions and avoiding the pitfalls.
Crossdisciplinary collaboration
The researchers in the team represent the KTH Schools of Engineering Sciences, the Department of Engineering Mechanics, the School of Industrial Engineering and Management, and the Climate Action Centre.
Watch the recorded presentation at the Digitalize in Stockholm 2023 event:
About the project
Objective
The aim of the research team is to realise and research the first objects of programmable, robotic matter. Robotic matter consists of thousands or millions micro-scale components and forms objects that can autonomously change their shape and material properties. The research results can be the foundation for future generations of robotic matters configured with various physical functionalities.
Background
Robotic matter does not exist today, but if we can create and use it, we could solve several major societal challenges. The ultimate scenario is the opportunity to create all kinds of objects. An everyday and relevant example is using robotic matter to create and re-create packaging instead of using non-circular plastic materials. Another example is a decreased demand for transportation of goods when we can create any object we want in the location where it is needed.
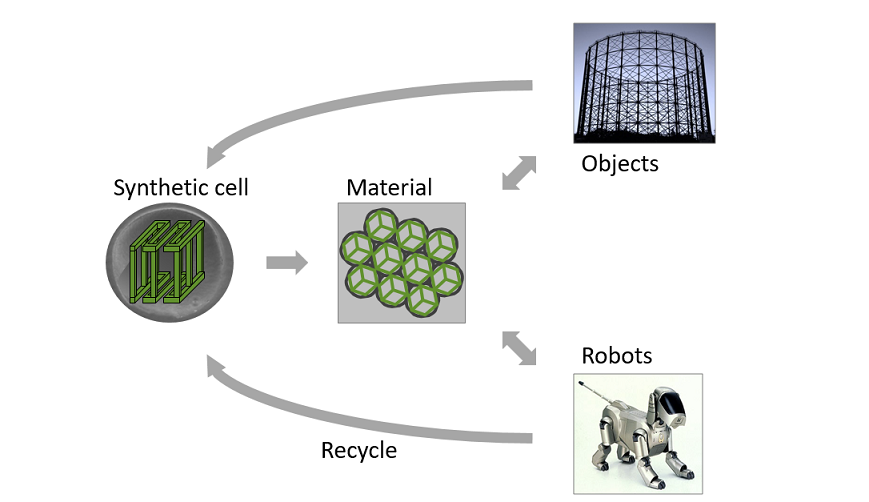
Cross-disciplinary collaboration
The research team represents the School of Electrical Engineering and Computer Science (EECS, KTH) and the School of Engineering Sciences in Chemistry, Biotechnology and Health (CBH, KTH).
Watch the recorded presentation at Digitalize in Stockholm 2022 event:
Press & Media
Article in Elektroniktidningen: https://issuu.com/etndigi/docs/etn2102ld
or download a pdf here: Article in Elektroniktidningen Feb 2021
Activities & Results
Find out what’s going on!
Activities, awards, and other outputs
To be announced
Results
We aim to build robotic matter consisting of thousands or millions of microscale components, so-called “synthetic cells”, that form objects that can autonomously change their shape and material properties.
Realising this objective requires combining four sub-objectives:
- Synthesis of the synthetic cells
- Thermoreversible bonding of the synthetic cells
- Magnetic orientation of synthetic cells with anisotropic properties to program the overall material properties emerging from the resulting cell conglomerate
- Transporting synthetic cell material throughout the object to program the overall material shape
Project results
WP1 Synthesis and thermoreversible bonding results:
Investigating the thermoreversible bonding of microparticles.
WP2 Magnetic material programming results:
Investigating integration of small magnets in every cell, and the material property programming by magnetically rotating the anisotropic cells prior to fixation.
WP3 Shape programming results:
Introducing shape programming of solid objects based on the local fluidisation of the object, followed by transport of the fluidised substance through the object by an internal pumping mechanism.
Publications
We like to inspire and share interesting knowledge!
- Programmable Matter with Free and High-Resolution Transfiguration and Locomotion; Kerem Kaya, Alexander Kravberg, Claudia Scarpellini, Emre Iseri, Danica Kragic and Wouter van der Wijngaart. First published on 24 December 2023 in Advanced Functional Materials.
- Copper-mediated synthesis of temperature-responsive poly(N-acryloyl glycinamide) polymers: a step towards greener and simple polymerisation; Nikola Křivánková, Kerem Kaya, Wouter van der Wijngaart and Ulrica Edlund. First published on 4 October 2023 in RCS Advances.
- Soft metamaterial with programmable ferromagnetism; Kerem Kaya, Emre Iseri and Wouter van der Wijngaart. First published on 6 December 2022 in Microsystems and Nanoengineering.
About the project
Objective
In the Data-Limited Learning of Complex Dynamical Systems (DLL) project, we develop methods and tools to learn to control complex dynamic systems using limited data samples. In contrast to traditional machine learning techniques that require large amounts of data for training, this project aims to utilize a priori knowledge of the system and combine such structural knowledge reliably with a limited number of data samples.
The project focuses on two application domains: (i) continuous bioprocessing for safer and more efficient production of biopharmaceuticals and (ii) reinforcement learning of cyber-physical systems in general and robotics in particular. In these domains, probing large amounts of data from the system can be expensive or even impossible without wearing out the system.
Background
Recent years have witnessed spectacular successes in applying machine learning tools to the decision-making and control of complex dynamical systems. These techniques typically combine reinforcement learning with large neural networks, thus requiring a tremendous amount of training data, even to learn simple tasks. Their applications have been mainly limited to specific scenarios, such as board and video games, where generating and gathering data is inexpensive. However, in many biological or physical application domains, data is limited, and probing the system for more data can be expensive or even impossible without destroying the system.
Cross-disciplinary collaboration
This project involves co-PIs and researchers from different disciplines, including computer science, automatic control, machine learning, and biotechnology. To make the interaction as fruitful as possible, the project is divided into three sub-projects: continuous bioprocessing, (ii) reinforcement learning in cyber-physical systems, and (iii) theory. The research involves fundamental theory and practical applications, including involvement from industrial partners. The collaboration between the research team at Digital Futures and the Competence Centre for Advanced BioProduction (AdBIOPRO) will establish a strong, visible, and sustainable research environment that will overarch digitalization and life science research at KTH.
Watch the recorded presentation at the Digitalize in Stockholm 2023 event:
Activities & Results
Find out what’s going on!
Activities, awards, and other outputs
- Tutorial at the IEEE International Conference on Signal Processing and Communications (SPCOM) 2022. Tutorial title: Generative models and role of deep neural networks. Lecturer: Saikat Chatterjee.
- Two-day Tutorial at Digital Futures on the Fundamentals of Bayesian Inference using Probabilistic Programming Probabilistic programming. Lecturer: David Broman.
- Distinguished Artifact Award at European Symposium on Programming (ESOP 2022), for the paper Compiling Universal Probabilistic Programming Languages with Efficient Parallel Sequential Monte Carlo Inference. David Broman and co-authors.
- Keynote at the Workshop on Nonlinear System Identification Benchmarks. Speaker: Håkan Hjalmarsson
- Organization of the Data-limited learning workshop (DLL) in fall 2021. Organized by David Broman together with Co-PIs.
- Organization of the MATH, AI, and Neuroscience (MAIN) workshop at Digital Futures in 2021. Organizer: Saikat Chatterjee
- Invited talk at the Stochastic Networks conference (Cornell in 2022). Speaker: Alexandre Proutiere
- Keynote at YEQTIV Eindhoven and in the SNAPP seminar series. Speaker: Alexandre Proutiere
- The organizer of the International Conference on Embedded Software (EMSOFT), the premier venue for embedded software, 2022. PC Chair: David Broman
Results
This project has so far resulted in several fundamental research results. The results include but are not limited to: a new biological modelling approach that includes transcriptional information, new system identification techniques for differential-algebraic equations subject to disturbances, fundamental limits for sample complexity and lower bounds for the regret of deterministic discrete dynamical systems.
The results are published in top-tier journals and conferences such as ICML, NeurIPS, and CDC.
Publications
- Filippo Vannella, Jaeseong Jeong, and Alexandre Proutiere. Off-Policy Learning in Contextual Bandits for Remote Electrical Tilt Optimization. IEEE transactions on Vehicular Technology, 2022.
- Filippo Vannella, Alexandre Proutiere, Yassir Jedra, and Jaeseong Jeong. Remote Electrical Tilt Optimization: a contextual bandit approach. In Proceedings of the IEEE International Conference on Computer Communications (INFOCOM) 2022.
- Daniel Lundén, Joey Öhman, Jan Kudlicka, Viktor Senderov, Fredrik Ronquist, and David Broman. Compiling Universal Probabilistic Programming Languages with Efficient Parallel Sequential Monte Carlo Inference. In the Proceedings of 31st European Symposium on Programming (ESOP), 2022.
- Kévin Colin, Mina Férizbegovic, and Håkan Hjalmarsson, Regret Minimization for Linear Quadratic Adaptive Controllers using Fisher Feedback Exploration, IEEE Control Systems Letters and In Proceedings of the 61th IEEE Conference on Decision and Control (CDC), 2022.
- Yassir Jedra and Alexandre Proutiere. Minimal expected regret in online LQR. In Proceedings of the International Conference on Artificial Intelligence and Statistics(AISTATS), 2022.
- Robert Bereza, Oscar Eriksson, Mohamed R.-H. Abdalmoaty, David Broman andHåkan Hjalmarsson. Stochastic Approximation for Identification of Non-Linear Differential-Algebraic Equations with Process Disturbances, In Proceedings of the IEEE Conference on Decision and Control (CDC), 2022.
- A Ghosh, A.E. Fontcurbeta, Mohamed R. Abdalmoaty, Saikat Chatterjee, Time-varying Normalizing Flow for Generative Modeling of Dynamical Signals, European Signal Processing Conference (EUSIPCO), 2022.
- S.Das, A.M. Javid, P.B. Gohain, Y.C. Eldar, S. Chatterjee. Neural Greedy Pursuit for Feature Selection, In the Proceedings of the International Joint Conference on Neural Networks (IJCNN), IEEE WCCI 2022.
- X Liang, AM Javid, M Skoglund, S Chatterjee, Decentralized learning of randomization-based neural networks with centralized equivalence, AppliedSoft Computing, 2022.
- David Broman. Interactive Programmatic Modeling. In ACM Transactions on Embedded Computing Systems (TECS), Volume 20, Issue 4, Article No 33, Pages 1-26, ACM, 2021.
- Mohamed R.-H. Abdalmoaty, Oscar Eriksson, Robert Bereza, David Broman and Håkan Hjalmarsson. Identification of Non-Linear Differential-Algebraic Equation Models with Process Disturbances, In Proceedings of the IEEE Conference on Decision and Control (CDC), 2021.
- Mina Ferizbegovic, Per Mattsson, Thomas Schön, and H. Hjalmarsson. Bayes Control of Hammerstein Systems, 19th IFAC Symposium on System Identification, 2021.
- Daniel Lundén, Johannes Borgström, and David Broman. Correctness of Sequential Monte Carlo Inference for Probabilistic Programming Languages. In Proceedings of 30th European Symposium on Programming (ESOP), LNCS vol. 12648, Springer, 2021.
- Viktor Palmkvist, Elias Castegren, Philipp Haller, and David Broman. Resolvable Ambiguity: Principled Resolution of Syntactically Ambiguous Programs. In Proceedings of the 30th ACM SIGPLAN International Conference on Compiler Construction (CC), ACM, 2021.
- A.M. Javid, S. Das, M. Skoglund and S. Chatterjee, A ReLU Dense Layer to Improve the Performance of Neural Networks, in Proceedings of the IEEE International Conference on Acoustics, Speech and Signal Processing (ICASSP), 2021.
- X. Liang, M. Skoglund, and S. Chatterjee, Feature reuse for a randomization based neural network, in Proceedings of the IEEE International Conference on Acoustics, Speech and Signal Processing (ICASSP), 2021.
- P.G. Jurado X. Liang, A.M. Javid, and S. Chatterjee, Use of deterministic transforms to design weight matrices of a neural network, in European Signal Processing Conference (EUSIPCO), 2021.
- Díogo Rodrigues, Mohamed R. Abdalmoaty, Elling W. Jacobsen, Véronqiue Chotteau, and Håkan Hjalmarsson. An Integrated Approach for Modeling and Identification of Perfusion Bioreactors via Basis Flux Modes, Computers & Chemical Engineering, 2021.
- Aymen Al Marjani and Alexandre Proutiere. Adaptive Sampling for Best Policy Identification in Markov Decision Processes. In Proceedings of International Conference on Machine Learning (ICML), 2021.
- Aymen Al Marjani, Aurélien Garivier, and Alexandre Proutiere: Navigating to the Best Policy in Markov Decision Processes. In Proceedings of the Conference on Neural Information Processing Systems (NeurIPS), 2021.
- Damianos Tranos and Alexandre Proutiere: Regret Analysis in Deterministic Reinforcement Learning. In Proceedings of the IEEE Conference on Decision and Control (CDC), 2021.
- Zhang, L., Wang, M., Castan, A., Hjalmarsson, H. and Chotteau, V., Probabilistic model by Bayesian network for the prediction of antibody glycosylation in perfusion and fed‐batch cell cultures. Biotechnology and Bioengineering, 118(9), pp.3447-3459, 2021.
- Chotteau, V., Hagrot, E., Zhang, L., Mäkinen, M. Mathematical modelling of cell culture processes. In Cell Culture Engineering and Technology (pp. 467-484). Springer, Cham, 2021.
- Mingliang Wang, Riccardo S. Risuleo, Elling W. Jacobsen, Véronique Chotteau and Håkan Hjalmarsson. Identification of Nonlinear Kinetics of Macroscopic Bio-reactions Using Multilinear Gaussian Processes, Computers and Chemical Engineering, 123(2), 2020.
- Stefanie Fonken, Mina Ferizbegovic, and H. Hjalmarsson. Consistent Identification of Dynamic Networks Subject to White Noise Using Weighted Null-Space Fitting, In Proceedings of the IFAC 2020 World Congress, 2020.
- Riccardo S. Risuleo and Håkan Hjalmarsson. Nonparametric Models for Hammerstein-Wiener and Wiener-Hammerstein System Identification, In Proceedings of the IFAC 2020 World Congress, 2020.
- Saranya Natarajan and David Broman. Temporal Property-Based Testing of a Timed C Compiler using Time-Flow Graph Semantics. In the Proceedings of the Forum on specification & Design Languages (FDL 2020), IEEE, 2020.
- Mohamed R. Abdalmoaty and Håkan Hjalmarsson. Identification of Stochastic Nonlinear Models Using Optimal Estimating Functions, Automatica, 119, 2020. Mina Ferizbegovic, Jack Umenberger, Håkan Hjalmarsson and Thomas Schön. Learning robust LQ-controllers using application oriented exploration, IEEE Control Systems Letters, 4(4):19-24 and jointly published in the Proceedings of the IEEE Conference on Decision and Control (CDC), 2020.
- A.M. Javid, A. Venkitaraman, M. Skoglund, S. Chatterjee, High-dimensional neural feature design for layer-wise reduction of training cost, EURASIP Journal on Advances in Signal Processing, 2020.
- Yassir Jedra and Alexandre Proutiere. Finite-time Identification of Stable Linear Systems Optimality of the Least-Squares Estimator. In Proceedings of the IEEE Conference on Decision and Control (CDC), 2020.
- Filippo Vannella, Jaeseong Jeong, and Alexandre Proutiere. Off-policy Learning for Remote Electrical Tilt Optimization. VTC-Fall, 2020.
- Jack Umenberger, Mina Ferizbegovic, Thomas Schön and Håkan Hjalmarsson. Robust exploration in linear quadratic reinforcement learning, In Proceedings of the Conference on Neural Information Processing Systems (NeurIPS), 2019 (Spotlight paper).
- Yassir Jedra, Alexandre Proutiere. Sample Complexity Lower Bounds for Linear System Identification. In Proceedings of the IEEE Conference on Decision and Control (CDC), 2019.
About the project
Objective
The goal of the collaborative impact project is to leverage the novel technical and theoretical research results from the data-limited learning project to create added value and technical transfer to the Swedish industry (Saab and AstraZeneca) and to create interactive physical demonstrators for the Digital Futures Hub and the general public.
Background
This impact project is an extension to the 4-year project Data-Limited Learning of Complex Dynamical Systems (DLL), where the new project focuses on impact activities, software prototypes, and demonstrators. See the following for the previous DLL project.
The previous DLL project consisted of three connected sub-projects (i) bioprocessing, (ii) reinforcement learning for cyber-physical systems, and (iii) theoretical foundation. Within these sub-projects, our project has resulted in significant research results. In this new collaborative impact project, we focus on a subset of these results within the three tasks. The key aspect of the usefulness of this new project is to take the results from a theoretical or pure academic setting, to create demonstrators for the public, and to enable technical transfer to the Swedish industry.
Cross-disciplinary collaboration
This project involves co-PIs and researchers from different disciplines, including computer science, automatic control, machine learning, and biotechnology. The project is divided into three main project tasks, each aiming for separate impact activities:
- Task 1: Prototype of tracking application with Saab. Here, we use a new theoretical framework for estimation resulting from the DLL project. The task concerns developing software prototypes together with Saab, to enable a defense system for tracking enemy drones, and to separate between different kinds of objects in space.
- Task 2: Interactive Humanoid Robot Demonstrator. We use both practical and theoretical results from the DLL project when developing an interactive and exciting demonstrator of a child humanoid robot, which will be on display at the Digital Futures Hub and showcased for the Swedish media.
- Task 3: Software for generalized bioprocess modelling and optimization. This project task aims to develop user-friendly software that uses a data-driven approach for the kinetic modelling of bioprocesses. The aim is for external partners, including AstraZeneca, to test the solution on their own cell lines and processes.
Objective
With the integration of information and communications technology and intelligent electric devices, substation automation systems (SAS) greatly boost the efficiency of power system monitoring and control. However, substations also bring new vulnerabilities at the frontier of a bulk power system’s wide-area monitoring and control infrastructure. They are known to be attractive targets for attackers. In this project, we will research, develop, and validate algorithms that defend against cyberattacks that aim to disrupt substation operations by maliciously changing measurements and/or spoofing spurious control commands.
We propose multiple use-inspired AI innovations that crucially leverage concurrent capabilities of SAS to transform the cyber security of power systems, including (i) a framework that synergizes optimization-based attack modelling with inverse reinforcement learning for multi-stage attack detection, (ii) a decision-focused distributed CPS modelling approach, and (iii) a mathematical program with equilibrium constraints framework of adversarial unlearning for spoofing detection.
Background
In the IEC 61850-based Substation Automation System (SAS), integrating computing and communication technologies with Intelligent Electric Devices (IEDs) greatly enhances the efficiency of power system monitoring and control. The fast-growing connectivity via wide area networks (WAN) enables powerful automation functions but also brings cyber vulnerabilities concerning new attack vectors. The substations are known to be attractive targets for attackers since they form the frontier of the wide-area monitoring and control infrastructure of a bulk power system, which consists of a Supervisory Control And Data Acquisition (SCADA) system, an Energy Management System (EMS), and a control centre.
Cyberattacks at SASs may be performed by maliciously changing measurements from IEDs and merging units (MUs) and/or spoofing spurious control commands for one or more switching devices from IEDs. An attack can alter a device’s configuration even if commands and data comply with syntax, protocol, and the targeted device. The vulnerabilities of the modern grid are many, as described in a National Academies Report.
Crossdisciplinary collaboration
Anomaly detection can reduce cyber threats to substations and improve root cause analysis. Traditional anomaly data detection heavily relies on human experts to design rule-based detection mechanisms, which can be time-consuming, inefficient, less adaptive, and labour-intensive. More recently, sophisticated anomaly detection methods have been reported in the literature. Still, they largely ignore the special characteristics of attacks on SAS and practical system-level constraints on communication and computation.
Transformative and disruptive applications of use-inspired AI for SAS anomaly detection are in their infancy. The proposed project is among the first known efforts to develop and demonstrate AI-enabled SAS anomaly data detection that crucially leverages the cross-disciplinary collaboration between substation Information engineering and Communications Technology (especially distributed machine learning) for cyber defence.
The project is a collaboration between the University of California Berkeley, Virginia Tech and KTH Royal Institute of Technology.
Watch the recorded presentation at the Digitalize in Stockholm 2023 event:
About the project
Objective
Soil carbon sequestration in croplands has tremendous potential to help mitigate climate change; however, it is challenging to develop optimal management practices to maximise the sequestered carbon and crop yield. This project aims to develop an intelligent agricultural management system using deep reinforcement learning (RL) and large-scale soil and crop simulations. To achieve this, we propose to build a simulator to model and simulate the complex soil-water-plant-atmosphere interactions, which will run on high-performance computing platforms.
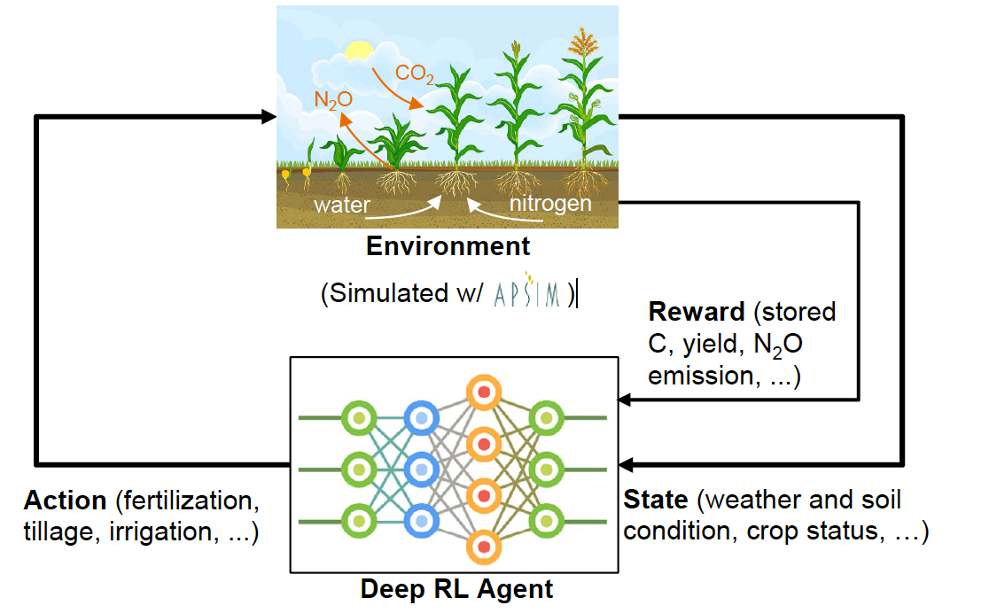
Background
Massive simulations using such platforms allow the evaluation of the effects of various management practices under different weather and soil conditions in a timely and cost-effective manner. By formulating the management decision as an RL problem, we can leverage the state-of-the-art algorithms to train management policies, which are expected to maximise the stored organic carbon while maximising the crop yield. The whole system will be tested using data on soil and crops in both the mid-west of the United States and the Mediterranean region. The proposed research has great potential to impact climate change and food security, two of humanity’s most significant challenges.
Crossdisciplinary collaboration
This project is a collaboration between the University of Illinois at Urbana-Champaign, KTH Department of Sustainable Development and Stockholm University, Department of Physical Geography.
Watch the recorded presentation at the Digitalize in Stockholm 2023 event:
About the project
Objective
In this project, Princeton University and KTH Royal Institute of Technology plan to develop a family of C3.ai-enabled methods for learning, optimization, and system stability analysis of grid-tied inverters and power electronics-based power systems. The goal is to develop a unified machine-learning platform for power electronics, power systems, and data science research. A bottom-up approach, from modelling a single inverter to modelling a cluster of inverters connected as a microgrid, will be used as a motivating case study to show a holistic hierarchical modelling approach supported by C3.ai.
Background
Distributed and renewable energy resources are massively deployed in electric power grids, driven by the sharp cost reduction and the demand for carbon neutrality. The grid of the future will be supported by clouds of distributed and renewable energy resources. Power electronics Inverters are pervasively needed to connect renewable energy resources to the grid, thanks to their full controllability over electric power. On the other hand, to meet a wide range of grid requirements, these grid-tied inverters are commonly equipped with sophisticated control systems, which pose challenges to the stability and control of power grids, threatening the energy security of modern society.
Crossdisciplinary collaboration
This project is a collaboration between KTH and Princeton University.
Watch the recorded presentation at Digitalize in Stockholm 2022 event: