About the project
Objective
The Enabling Machine-Learning Intelligence for Network Cybersecurity (EMERGENCE) aims at enabling machine-learning-based analysis of high-speed network cybersecurity data. The first part of the project focuses on extracting the relevant fine-grained network metrics directly in the network devices and transforming these collected metrics into summaries that can be easily extracted from the devices. The second part of the project takes these summaries and feeds them into a machine learning system that is tailored to detect security attacks and performance-related issues. A key idea in the project is to leverage programmable network technologies that allow performing ad-hoc operations at the speed of the network before the summaries are sent to the slower machine learning systems.
One of the envisioned contributions of the project is the design and implementation of a framework that reconciles the different speeds at which today’s networks and machine learning systems operate.
Background
During the current global pandemic crisis, the Internet has played an essential role in allowing different parts of our society to continue operating without interruptions to the largest extent possible. However, the recent wave of cyber-attacks targeting the Internet infrastructure has raised concerns about the resilience of the Internet infrastructure. In contrast to general cybersecurity threats, which affect end-host systems, Internet-based network attacks target the core infrastructure of the Internet that is responsible for interconnecting all the billions of users, devices, and services together. Machine learning techniques to detect network-based cyber-attacks have long been limited by two unique aspects of the networking domain. First, network data is inherently volatile as traffic flows through a network without being stored. Second, network technologies are ill-suited for extracting fine-grained network information from high-speed networking devices. Both challenges will be addressed by relying on the emerging high-speed programmable network devices.
Crossdisciplinary collaboration
The researchers in the team represent the School of Electrical Engineering & Computer Science, KTH, and the Connected Intelligence unit at RISE Research Institutes of Sweden.
Watch the recorded presentation at Digitalize in Stockholm 2022 event:
About the project
Objective
SHIFT-DT aims to transcend the current advancements in ship design and production technologies by establishing a framework that incorporates the decarbonization of shipping by marrying holistic ship design with digitalized ship production and logistics through the application of digital twins based on the model-based systems engineering method. This novel approach positions digital twin technology as the next-generation solution for sustainable ship design and production. By realizing these research objectives, we can contribute significantly to integrating digital and innovative solutions in sustainable ship design and production.
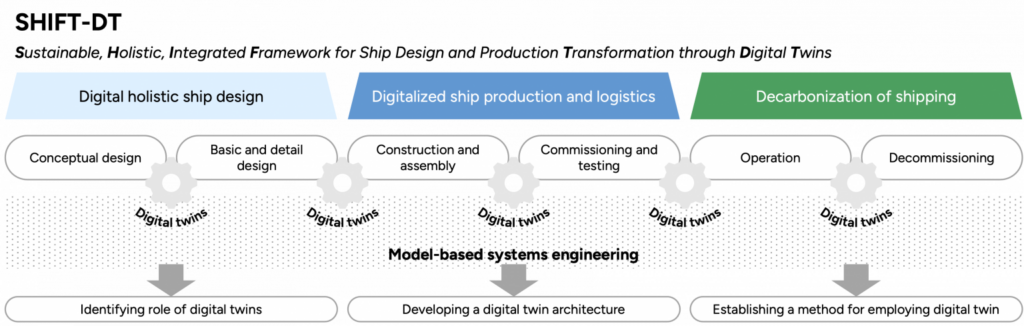
Background
Digitalization and decarbonization are the transformative forces that will shape the future of shipping. Through technologies like Digital Twins (DTs), digitalization has the potential to revolutionize ship design and production, thereby advancing decarbonization. Extensive literature reviews, however, suggest that the application of DTs in ship design and production is nascent. Studies involving ships designed entirely with DTs are notably lacking, presenting the maritime industry with the significant challenge of establishing a new design methodology for ships using DT technology. This challenge extends to the broader manufacturing industry, where new design processes are evolving slowly and implementing DTs in ship production is an emerging, complex concept.
Crossdisciplinary collaboration
A collaboration has been established between the KTH Center for Naval Architecture at the Department of Engineering Mechanics and the Production Logistics Research Group at the Department of Production Engineering. This multidisciplinary team, comprising recognized experts in ship design and production, is exceptionally positioned to lead the digitalization of sustainable ship design and production.
About the project
Objective
In Emergence 2.0, we aim to build reliable, secure, high-speed edge networks that enable the deployment of mission-critical applications, such as remote vehicle driving and industrial sensor & actuator control. We will design a novel system for enabling fine-grained network visibility and intelligent control planes that are i) reactive to quickly detect and react to anomalies or attacks in edge networks and IoT networks, ii) accurate to avoid missing sophisticated attacks or issue unwanted alerts without reason, iii) expressive, to support complex analysis of data and packet processing pipelines using ML classifiers, and iv) efficient, to consume up to 10x less energy resources compared to state of the art.
Background
Detecting cyber-attacks, failures, misconfigurations, or sudden changes in the traffic workload must rely on two components: i) an accurate/reactive network monitoring system that provides network operators with fine-grained visibility into the underlying network conditions and ii) an intelligent control plane that is fed with such visibility information and learns to distinguish different events that will trigger mitigation operations (e.g., filtering malicious traffic).
Today’s networks rely on general-purpose CPU servers equipped with large memories to support fine-grained visibility of a small fraction (1%) of the forwarded traffic (i.e., user-faced traffic). Even for such small amounts of traffic, recent work has shown that a network must deploy >100 general-purpose, power-hungry CPU-based servers to process a single terabit of traffic per second, costing millions of dollars to build and power with electricity. Today’s data centre networks must support thousands of terabits per second of traffic across their cloud and edge data centre infrastructure.
Crossdisciplinary collaboration
The researchers in the team represent the KTH School of Electrical Engineering and Computer Science, the Department of Computer Science and the Connected Intelligence unit at RISE Research Institutes of Sweden.
Watch the recorded presentation at the Digitalize in Stockholm 2023 event:
About the project
Objective
In collaboration with Karolinska University Hospital (KUH) and Karolinska Institute (KI), the PI and Co-PI of KTH propose the ISPP postdoc project EMERDENSY to develop trust-worthy machine learning algorithms with explainable outcomes and then use the algorithms for the design of Early Warning Systems (EWS).
Background
Artificial Intelligence (AI) can be used to detect infection. Often, doctors and nurses cannot be sure about the growth of infection due to the absence of clearly visible symptoms. Once infection starts, the body’s immune system starts to fight bacteria and viruses. Physiological parameters of the body, such as heart rate, blood pressure, breathing patterns, and temperature, change slowly.
AI can detect subtle changes, but humans cannot. AI can also predict infection type and patient deterioration. The medical care team will then spend precious time deciding on life-saving interventions. This heralds the use of AI-based early warning systems (EWS).
The big question: can we trust the AI systems, mainly its core called machine learning for data analysis and predictions? Can the machine learning algorithms explain their predictions to the healthcare personnel?
Partner Postdoc(s)
Yogesh Todarwal
Main supervisor
Saikat Chatterjee, Associate Professor, Division of Information Science and Engineering at KTH
Co-supervisor
Sebastiaan Meijer, Professor and Vice Dean, Division of Health Informatics and Logistics at KTH
Watch the recorded presentation at the Digitalize in Stockholm 2023 event:
About the project
Objective
The PERCy project aims to develop a reference architecture, procedures and algorithms that facilitate advanced driver assistance systems and ultimately fully automated driving by fusing data provided by onboard sensors, off-board sensors and, when available, sensory data acquired by cellular network sensing. The fused data is then exploited for safety-critical tasks such as manoeuvring highly automated vehicles in public, open areas. This framework is motivated by the key observation that off-board sensors and information sharing extend the safe operational design domain achieved when relying solely on on-board sensors, thus promising to achieve a highly improved performance-safety balance.
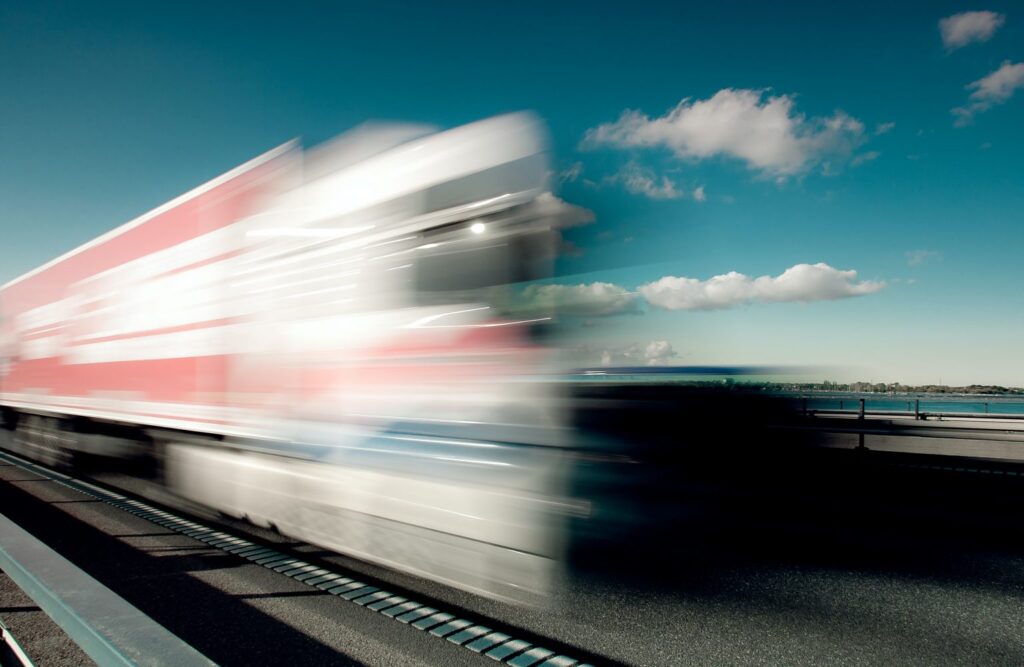
Background
Advanced driver assistance systems – such as adaptive cruise control, autonomous emergency braking, blind-spot assist, lane keep assist, and vulnerable road user detection – are increasingly deployed since they increase traffic safety and driving convenience. These systems’ functional safety and general dependability depend critically on onboard sensors and associated signal-processing capabilities. Since advanced driver assistance systems directly impact the driver’s reactions and the vehicle’s dynamics and can cause new hazards and accidents if they malfunction, they must comply with safety requirements. The safety relevance of onboard sensors is even higher in the case of highly automated driving, where the human driver does not supervise the driving operation. However, current standards and methodologies provide little guidance for collaborative systems, leading to many open research questions.
Crossdisciplinary collaboration
This project is a collaboration between KTH, Ericsson and Scania.
Watch the recorded presentation at the Digitalize in Stockholm 2023 event:
About the project
Objective
Atlas Copco is a world leader in safety-critical assembly tools widely used in industries like automotive, aviation, and mobile phones. To maintain its world-leading position, Atlas Copco would like to error-proof its handheld tightening tools to ensure that depending on the assembly, the bolts are tightened with the right tightening program and in the right sequence, and no bolts are left untightened. Atlas Copco would like to use an AI/ML-based sensor fusion system to achieve this. The form factor, power budget, latency, and cost requirements rule out the use of COTS like GPUs, TPUs, or FPGAs. ASICs (Application Specific Integrated Circuits) would meet these constraints, but they take too long, are very expensive, and require specialist competence that Atlas Copco lacks. KTH has developed a Lego-inspired design framework called SiLago that provides ASIC-comparable energy efficiency and form factor but allows non-specialists like mechatronic and AI/ML experts from Atlas Copco to create a ready-to-manufacture custom chip.
The objective of this demo project is to validate the claim of SiLago by showing that it can be used by non-specialists to implement this challenging AI/ML system and meet its constraints.
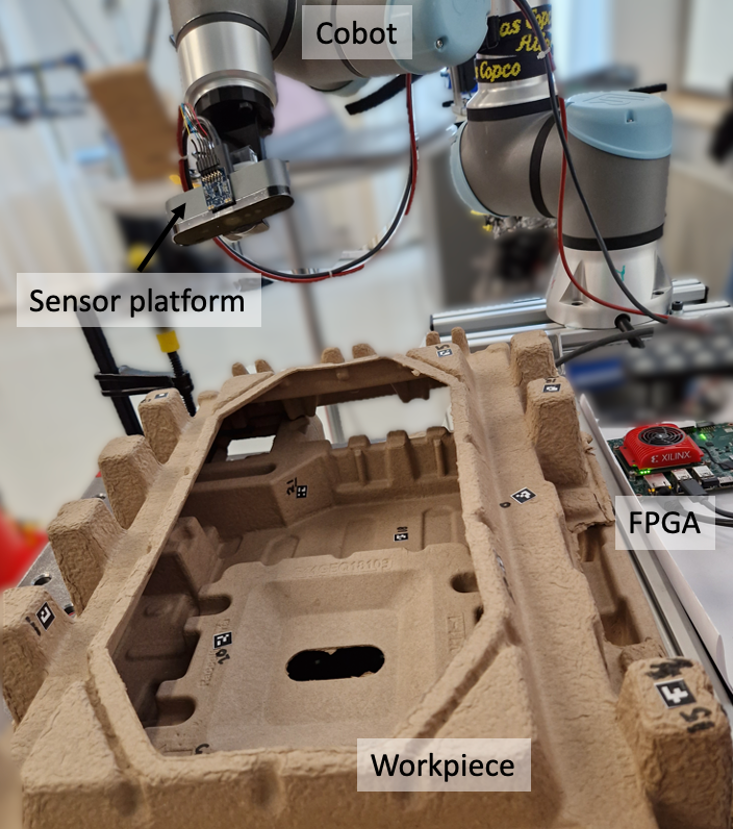
Background
Industry 4.0 or the Fourth Industrial Revolution refers to a significant transformation in how manufacturing and other industries operate, characterized by the integration of digital technologies, automation, data exchange, and smart systems. To align its product offerings with the Industry 4.0 vision, Atlas Copco has launched a pilot project to error-proof its tightening tools to improve the assembly process’s reliability, flexibility, and productivity. To achieve these objectives, it is developing an AI/ML-based sensor fusion system. Energy and cost-efficient implementation of such systems is a well-recognized challenge, and many large actors like Tesla, Google, etc. have opted for an ASIC-based design. However, the design cost of ASICs runs in 100s of MUSDs and requires large volumes and/or large profits to justify them. This often restricts ASICs as a solution to large actors.
To democratize access to ASIC-like efficiency for small actors and non-specialists, KTH has proposed a Lego-inspired design framework called SiLago. The impact of this demo project will be well beyond this specific use case from Atlas Copco; it will open the doors for ecologically and economically scalable energy-efficient digitalization in many sectors, including scientific super-computing.
Crossdisciplinary collaboration
This demo project spans multiple disciplines: mechatronics, AI and ML algorithms, sensor informatics, computer architecture, and VLSI design. It is a collaboration between KTH and Atlas Copco.
About the project
Objective
The project aims to develop an integrated digital infrastructure system to enhance the level of automation for smart construction. The initial goal will involve the creation of models for the digital twin of the robotic environment on construction sites. The digital twin will be used for remote real-time monitoring, prediction, optimization and multi-robot task planning and control. The results will be tested and applied to a practical Skanska use case.
Background
Construction sites today still rely to a large amount on manual labour, and the vision for the future is to leverage automation equipment (machines, robots) to the largest extent possible in order to speed up the production cycle, enhance quality while also reducing human risks, carbon emissions and costs. Smart construction, in essence, a flexible automation process, requires a stringent digitalization of the construction site in terms of real-time digital twins of products and production systems paired with advanced algorithms for the control of machines, the coordination of robots and the assurance of safety at the workspace.
The project strives to realise such systems, leading to fundamental research challenges and practical implementations in relevant use cases. The project developments can lay the foundation for future activities by forming and evolving a consortium nucleus.
Crossdisciplinary collaboration
The project partners are Ericsson AB, Skanska and KTH.
Watch the recorded presentation at the Digitalize in Stockholm 2023 event: