About the project
Objective
Aligning with the Digital Futures call for a “rich and healthy life,” this research will explore designing for collective caring as the core of the next generation Femtech for menstrual and reproductive wellbeing. Using a cross-cultural approach (Sweden and India), the objective is to investigate what it means to nurture trust and cooperation when engaging menstruators with parents and/or partners to digitalize collective caring for intimate health. Here, it is crucial to acknowledge that the conventional methodological approach to participant recruitment and data collection inadequately addresses the stigma and language barriers inherent in the taboo nature of the topic.
Thus, using a qualitative method and participatory design approach, this research will explore assets of culture and heritage for crafting appropriate methods and designing Femtech to navigate taboos and build trust for facilitating a dialogue on the sensitive topic of intimate health and wellbeing. The motivation to undertake this research stems from a commitment to fostering equitable and period-positive futures where menstruating bodies are not stigmatized or seen as needing control, discipline, or fixing.
Background
Menstruation is a biological phenomenon experienced by millions of individuals daily around the globe, where varied stakeholders in a menstruator’s vicinity shape these experiences. Throughout the reproductive journey (menarche to menopause), parents and partners play pivotal roles in a menstruator’s life. In certain scenarios and contexts, the decision-making and/or financial power may be disproportionately in the hands of these two stakeholders. Thus, focusing on menstruators’ intimate health and well-being, it becomes imperative to expand the contemporary personal informatics approach to design Femtech by taking an ecological approach focusing on interpersonal cooperation to ideate, design, and implement Femtech.
About the Digital Futures Postdoc Fellow
As a qualitative researcher and interaction designer, Anupriya Tuli focuses on understanding and designing technology experiences toward just and equitable futures. Her research is situated at the intersection of Human-Computer Interaction (HCI), global health, taboo subjects, marginalization, and activism. She frequently engages with feminist perspectives, aiming to design socially responsible technologies that target health equity, focusing on women’s health, well-being, and empowerment.
She completed her PhD in Human-Centered Design at IIIT-Delhi, India, where she delved into the design of digital menstrual technologies to uncover how technology design can effectively address cultural taboos and systemic barriers to nurture positive menstrual experiences. She received fellowships from the Indian Government and Google to support her doctoral research. In addition to her academic endeavours, she is deeply involved in collaborative efforts with practitioners and non-governmental organizations in India, particularly focusing on initiatives related to menstrual health and well-being.
Main supervisor
Madeline Balaam, Professor, Division of Media Technology and Interaction Designs at KTH Royal Institute of Technology
Co-supervisor
Airi Lampinen, Associate Professor, Department of Computer and Systems Sciences (DSV) at Stockholm University
About the project
Objective
This research will develop design considerations and policy recommendations for designing with and applying (inter)personal and intimate data. The project will follow a participatory approach centered around people’s experiences interacting with and sharing intimate technologies that collect and store (inter)personal data.
Background
In today’s digital society, most people routinely interact with connected products and services that collect and indefinitely store personal data. Increasingly, these products and services — and the data they produce — permeate intimate spaces, such as smart vibrators collecting sensor data about people’s arousal and orgasm, and AI romance chatbots collecting self-reported information about people’s mental and sexual health. Moreover, these intimate spaces are often shared and relational. For instance, a connected voice assistant in a shared household collects data from the primary user, other household members, and even occasional visitors. Thus, data becomes both intimate and (inter)personal, shaped by and shared across (inter)personal relationships around shared experiences and spaces.
These characteristics raise critical questions for data, design, and policy. Although connected intimate technologies — and the data they produce — are often designed for individual use(rs), their use is often shared and relational: How can we design intimate technologies that empower their users to care for and share their data? Similarly, regulations such as the GDPR established several rights to empower individuals to control their data, such as the right to access: Who should access (inter)personal data?
About the Digital Futures Postdoc Fellow
Alejandra Gómez Ortega is a Design and Human-Computer Interaction researcher. She holds a PhD in Industrial Design Engineering from the Delft University of Technology in The Netherlands. Her research investigates individual experiences interacting with and sharing intimate data, privacy perceptions and considerations around data, and data themselves through playful and creative approaches. Alejandra has applied various methods and approaches in her design research journey, including Participatory Design and Research through Design. Alejandra enjoys designing, developing, deploying, and exhibiting provocative artifacts and digital prototypes that enable individuals and communities to experience a specific situation as a starting point for reflection and discussion.
Main supervisor
Airi Lampinen, Associate Professor, Department of Computer and Systems Sciences (DSV), Stockholm University.
Co-supervisor
Madeline Balaam, Professor, Division of Media Technology and Interaction Designs, KTH.
About the project
The SHARCEX project focuses on improving underwater operations by integrating advanced autonomous underwater vehicles (AUVs) with human divers. The goal is to enhance safety and efficiency in extreme underwater environments such as defense, rescue operations, and law enforcement.
Key technologies being developed and integrated include:
- Embedded AI for real-time decision-making
- Machine learning for environmental adaptation
- Computer vision for precise navigation and object recognition
- Safe reinforcement learning to ensure strict safety protocols
The project progresses through multiple phases:
- Development of AI models
- System integration
- User interface design
- Operational testing
KTH leads the project in collaboration with FMV and Saab, aiming to demonstrate robust AUV-Diver collaborations validated through extensive simulations and experiments.
Background
Underwater environments are among the most challenging operational domains due to their unpredictability, extreme pressure conditions, limited visibility, and communication constraints. Human divers working in these environments face significant safety risks, particularly in defense, search-and-rescue, law enforcement, and infrastructure inspection scenarios. While Autonomous Underwater Vehicles (AUVs) have been deployed in these fields, their potential remains largely untapped due to limitations in real-time adaptability and human interaction.
Current AUV systems often operate independently, following pre-programmed missions with limited real-time decision-making capabilities. This restricts their usefulness in dynamic and high-risk situations, where divers must quickly adapt to changing conditions, assess threats, and make complex decisions. There is a clear need for AUVs that can function as intelligent, real-time assistants, enhancing human capabilities rather than merely executing pre-set tasks.
The SHARCEX project addresses this gap by developing a next-generation human-robot collaboration frameworkfor underwater operations. By integrating AI-driven AUVs with human divers, the project aims to create a synergistic system where both human and machine leverage each other’s strengths.
Crossdisciplinary collaboration
The project integrates expertise from multiple fields:
- Artificial Intelligence & Machine Learning (real-time decision-making and reinforcement learning)
- Underwater Robotics & Control Systems (AUV operation and sensor integration)
- Human-Robot Interaction (enhancing collaboration between divers and robots)
- Computer Vision (object recognition and navigation)
- Safety & Risk Management (ensuring compliance with safety protocols in extreme environments)
Collaboration partners:
- KTH (lead institution, technical development, and validation)
- FMV (defense applications and operational requirements)
- Saab (industrial expertise in autonomous systems and marine technology)
Principal Investigators (PIs)
- Ivan Stenius (KTH, Project Lead)
- Jana Tumova (KTH co-PI)
- Dimos Dimarogonas (KTH co-PI)
About the project
Objective
With the OrganoFeed project, we aim to leverage our joint expertise regarding microfluidic engineering & integration, and predictive algorithms development, to help address a core problem in biomedical research: reproducibility. Specifically, we aim to greatly reduce the variability of organoid cultures, which otherwise hold great promise for improving both fundamental research and drug development, by shifting the paradigm from a homogenous chemical environment to individualized, data-driven feedback control.
Background
Organoids are miniaturized, self-assembled, and self-organized cellular constructs. They can recapitulate key morphology, cellular composition, and biological function of human organs, improving greatly upon the simplistic mono-cellular models in use for early drug development. At the same time, organoids’ human origin avoids the species mismatch inherent to animal testing, which currently contributes significantly to poor translatability from drug candidates to human clinical trials (not to mention inherent ethical concerns). Last but not least, being derived from individual human donors’ cell samples, organoids can be used to model both fully personalized response as well as true population-level sampling. Organoids are, however, sensitive to even small variations in their culture conditions over the often weeks-long course of their maturation, resulting in high variability of morphology, cell composition, and function.
Current mitigation approaches have focused on providing more homogenous conditions. We propose instead an entirely different approach, based on feedback-driven control of the chemical environment at the level of each individual organoid. This ability to generate highly homogenous organoid populations should further increase organoids’ attractiveness in replacing both overly simplistic cell models as well as ethically and functionally suspect animal models with something more meaningful.
Crossdisciplinary collaboration
In this project, we are establishing a new cross-disciplinary and complementary collaboration:
- Ioanna Miliou, Assoc. Prof., is an expert in data analysis and modeling, with proficiency in advanced statistical methods, machine learning, and predictive modeling, enabling the extraction of meaningful insights from complex datasets.
- Thomas E Winkler, Assoc. Prof., is an expert in microsystems integration for biomedical applications, such as organs-on-chips, with a focus on electrochemical sensors, microfluidic materials, and human-relevant cells or samples.
- Karolinska Institutet Stem Cell Organoid (KISCO) facility will lend its expertise regarding a range of cutting-edge organoid models and culture methods.
About the project
Objective
RECOPS aims to provide evidence-based insights into the benefits of open-source software in the power sector. The project focuses on identifying impactful areas for open-source applications, such as HVDC control, distributed renewable energy (DER) simulation, and hardware operations. Through case studies and a novel assessment methodology, RECOPS will evaluate system robustness and cost benefits from open-source approaches. The project is structured into three work packages, incorporates stakeholder engagement, simulations, and interdisciplinary collaboration.
Background
Open-source software has gained widespread use in industries like robotics and general software engineering but remains underutilized in the power sector. While academic initiatives exist, their limited maintenance and lack of industry relevance hinder broader adoption. The power sector’s need for robustness and focus on proprietary, closed software solutions further constrain open-source integration. However, there could be benefits with open-source software to enhance cost-effectiveness and robustness in energy systems.
Crossdisciplinary collaboration
The researchers in the team represent the KTH School of Industrial Engineering and Management, Unit of Energy Systems and the KTH School of Electrical Engineering and Computer Science, Division of Electric Power and Energy Systems.
About the project
Objective
This project aims to develop a Risk Prediction Tool to use as an early warning system in primary care, aimed at cancer using Colorectal Cancer as the first test case. The tool will be based on data collected at primary care units, such as care visits such as history, symptoms, medication, lab tests and results, as well as free text from physician notes. The tool will use machine learning (ML), large language models and advanced statistical analyses to assess the risk of patients having an underlying yet undiagnosed CRC. Despite the promise and potential of primary care data to assess risk and improve screening, very few studies use this potential to better inform diagnostics.
Existing risk assessment tools designed for use in primary care have been developed and validated with a limited dataset (ICD-10 codes that were present in primary care datasets, covering a smaller percentage of patients), but are not yet in clinical use. The project aims to gain an understanding of the most salient data points for assessing risk of CRC in primary care, as well as insight into the potential benefits of such analysis for the healthcare system. Through this analysis, the project also aims to obtain a validated, novel risk prediction tool for CRC, which can be widely deployed and feed into national guidelines for colorectal cancer. By using CRC as the initial test case, the project aims to develop methods and tools through the results of the project which can be modified for other cancers and applications.
Background
A majority of patients with cancer present with early symptoms in primary care, before receiving their diagnosis. Despite the fact that Sweden has fast track for cancer diagnosis since 2015 (cancer care pathway, CCP) primary care physicians lack decision support to assess cancer risk for individual patients. Colorectal cancer (CRC) is the third most common cancer in Sweden. Despite screening, most patients with CRC are diagnosed after symptom presentation. The survival is excellent in patients diagnosed with CRC limited to the bowel wall (stage I-II) and intermediate when it has spread to regional lymph nodes (stage III) while the prognosis is poor in the approx. 25% who are diagnosed with CRC with distant metastases (stage IV).
Cross-disciplinary collaboration
The project is a highly inter-disciplinary collaboration between KTH, Regional Cancer Centrum Stockholm-Gotland, Regional Cancer Centrum Väst and Karolinksa Institutet. The project team comprises primary care clinicians, statisticians, modelers, software engineers and planners at RCC.
About the project
Objective
AsthmaTuner is an existing remote digital tool owned by MediTuner AB in Sweden. AsthmaTuner consists of a handheld spirometer connected to a mobile app that can be used by patients with asthma and other respiratory diseases to diagnose and manage their health condition.
Explainable Artificial Intelligence (XAI) has high potential to enhance existing digital tools previously developed without AI or with black-box AI. The existing AsthmaTuner system does not use any AI so far, and naturally the scope of using black-box AI has a high potential. In this proposed project, we pursue a significant step ahead. We will explore how explainable AI (XAI) will enhance both the diagnostic and management capabilities of the AsthmaTuner system. That means, XAI will help both patient users and their health care providers further improve asthma care, with the target of reduced need for unnecessary health care visits, or costly and potentially deadly asthma attacks or exacerbations. The XAI-based digital tools are expected to prevent further health disparities and will include the addition of social determinants of health to the design, implementation, and evaluation to AsthmaTuner to improve the performance.
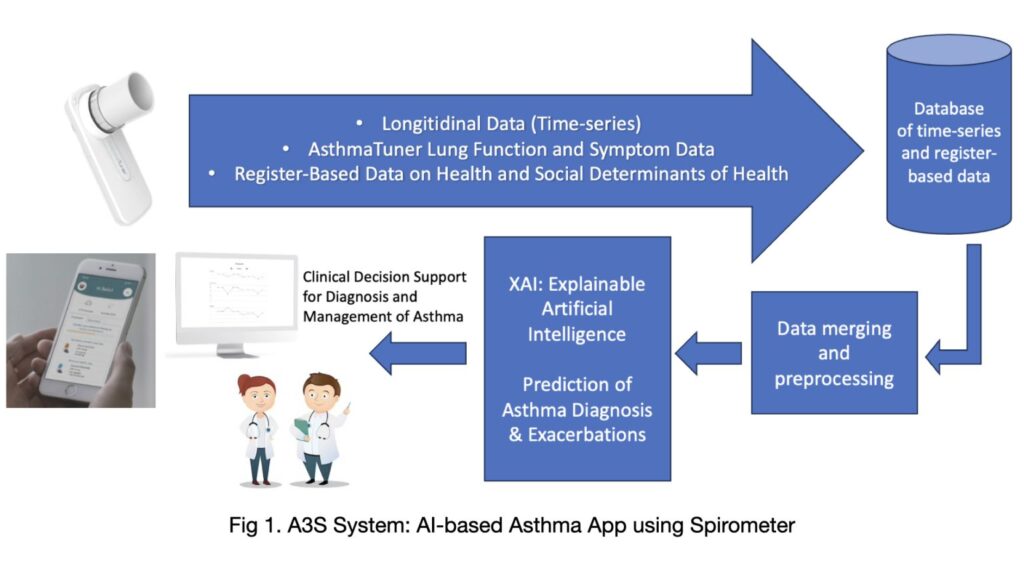
Background
Asthma is a large-scale health care problem, affecting around 10% of the European population, and moreover; asthma and other respiratory diseases are increasing due to environmental deterioration worldwide. Asthma is a complex problem, which is characterized by airway inflammation and respiratory symptoms of wheezing, dyspnea, chest tightness and cough that vary over time and in intensity, together with variable expiratory airflow limitation. Potentially effective ways to address asthma care are to better understand the day-to-day lung function (a longitudinal data) and asthmatic symptoms of individuals and more effectively identify, diagnose, and manage asthma by exploring potential disparities in symptoms, lung function, and outcomes that may exist by social determinants for patients. AsthmaTuner (AT) is the existing, validated digital tool in this regard.
The general purpose of the project is to design an explainable AI (XAI)-based smart AT system that uses a mobile app and a handheld spirometer. We refer to the proposed project as “A3S: AI-based Asthma App using Spirometer”. For the A3S system development, XAI algorithms will perform patient data analysis and guide decisions. The term “explainable” in XAI refers to the ability of these algorithms to provide transparent and interpretable explanations to patients and health care professionals for their predictions or decisions. The XAI algorithms will provide person-centred interventions precluding the onset or minimizing the risk of asthma in disadvantaged groups. It will help to improve respiratory health by training person-centred AI-support in asthma diagnosis and management of asthma in disadvantaged groups based on social determinants. Overall, the A3S project addresses a pressing health care problem with potentially widespread impact.
Cross-disciplinary collaboration
This is a highly collaborative project with a team of data scientists, clinicians, and AI specialists. Partners are PI Magnus Jansson, Co-PIs Saikat Chatterjee, PostDoc Zhendong Wang of EECS KTH, Ioanna Miliou of DSV SU, Docent Björn Nordlund of KUH and KI and his team, along with MediTuner AB.