About the project
Objective
GPARSE aims to mitigate the harm of collisions on road intersections proactively and reactively using edge infrastructure. The proactive approach orchestrates traffic arrival at the intersection using a novel concept of safety zones to avoid collisions. If a collision is nevertheless likely, the reactive approach enables the roadside unit to provide resources to the affected vehicles. This includes contingency path planning considering the safety zones and aggregated sensor data of the intersection for a complete view that cannot be obtained by the vehicles’ sensors alone. To achieve this, GPARSE will focus on nominal and contingency path planning, considering the novel concept of safety zones and the available information the roadside unit provides. This must be done to guarantee timely interaction between the different actors at the intersection. Therefore, GPARSE will develop techniques to allow the roadside unit to provision computation resources for the different types of workloads that emerge from sensors, pro-active tasks and reactive tasks so that the different timing constraints of the workloads are met. This is complicated by these constraints, often spanning across several chains of different computation tasks and platforms.
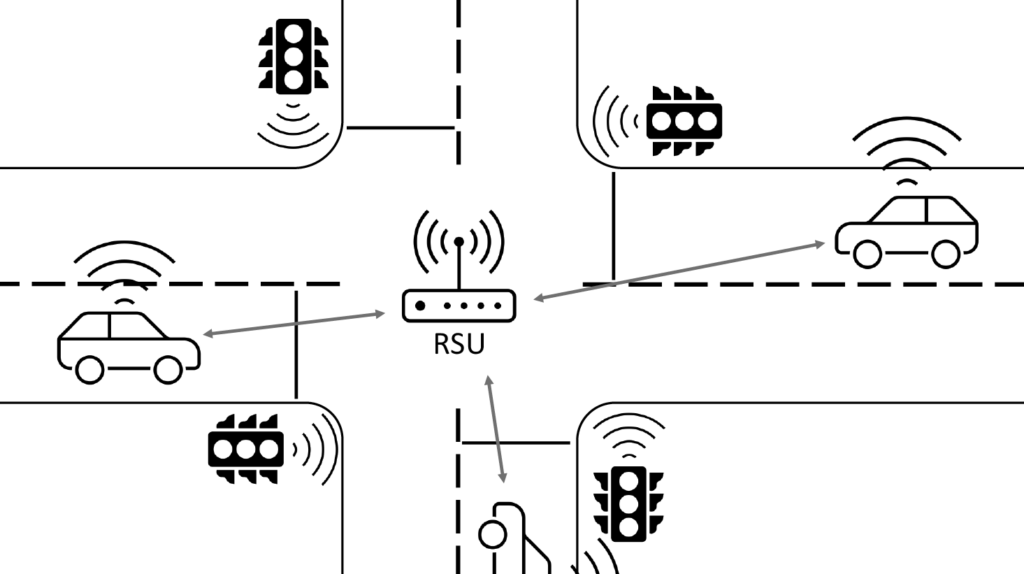
Background
Low-speed collisions at intersections are common but can still lead to permanent impairment – in particular for women. Rudimentary driver assistance systems can reduce the severity of injuries by braking, but more advanced mitigation of unavoidable collisions faces several challenges. In particular, no single vehicle has the necessary overview, other traffic that interferes with the situational awareness of driver assistance systems and can be involved in secondary collisions, and the short timeframes involved. Contingency path planning, i.e. the reconfiguration of unavoidable collisions to decrease the resulting harm, must be able to estimate the collision parameters of the involved actors at runtime. This is challenging in the general case and has a large effect on the outcome of the approach. At the same time, computation platforms on vehicles, as well as roadside units, must schedule heterogeneous workloads in such a way that diverse timing constraints are met. Therefore, online orchestration of dynamic and static workloads under temporal constraints across all compute nodes is necessary.
Crossdisciplinary collaboration
The researchers in the team represent the School of Engineering Engineering and Computer Science (ECS), KTH and the School of Industrial Engineering and Management (ITM), KTH.
About the project
Objective
The project aims to tackle several methodological and technical challenges encountered during the first phase of developing digital twins (DT) of the personalized human neuromusculoskeletal system: durable biomass sensor design, real-time modelling, and multiple sensor fusion. Overcoming the abovementioned bottlenecks will vastly improve the reliability and robustness of the DT framework. It can then be a clinical-friendly biofeedback neurorehabilitation platform based on a highly modularized and robust wearable sensor-fusion framework, including the innovative biomass-based ultrasound transparent electromyography electrodes.
Background
It is estimated that 15% of the world’s population lives with one or more disabling conditions. Impaired motor function is one of the major disabilities. Management of a complex disability currently largely relies on rehabilitation. In clinical practice, the supervision and the evaluation of a rehabilitation motion pattern remain a medical and engineering challenge due to the lack of biofeedback information about the effect of the rehabilitation motion on individual human biological tissues and structures. Digital twins (DT) is one of the most important concepts in digitalization, integrating all data, models, and other information that allows us to monitor the current states of a real system, e.g., a human musculoskeletal system in the current context. Among others, reliable and wearable sensor data fusion is critical in accomplishing the workflow of DT. The recent development of epidermal electronics offers a promising alternative. In particular, natural wood-derived nanocellulose shows promise in epidermal electronics for simultaneous dual signals collection due to biocompatibility, excellent mechanical properties, high water retention, and great potential for multi-functionalization.
Crossdisciplinary collaboration
This project brings expertise within biomechanical modelling, medical imaging, artificial intelligence and wood nanoscience, wood nanoengineering, and biomaterials design, involving researchers from the Department of Engineering Mechanics at KTH SCI and the Department of Fibre and Polymer Technology at KTH CBH.
About the project
Objective
DeepFlood aims to develop novel hybrid models and flood maps with water depth information to support real-time decision-making and present them to the Swedish and international scientific society and the stakeholders’ community. The research will be helpful for improving our fundamental understanding of SAR-based flood mapping by developing novel hybrid PolSAR-metaheuristic-DL models.
Background
Precise and fast flood mapping will help water resources managers, stakeholders, and decision-makers in mitigating the impact of floods. Rapid detection of flooded areas and information about water depth are critical for assisting flood responders, e.g., operation specialists, local and state authorities, etc., and increasing preparedness of the broader community through actions such as home risk mitigation and evacuation planning.
This project seeks to fill current knowledge gaps in flood management by enabling accurate and rapid flood mapping and providing water depth information using novel hybrid PolSAR-metaheuristic-DL models and high-resolution remote sensing data. It will also advance flood detection and support notification systems by identifying 1) bands and polarizations that contain the most information for detecting flooded areas in different land covers; 2) the most effective PolSAR features in each band for flood mapping; 3) whether the most informative PolSAR features are the same for different land covers; and 4) which of the widely used metaheuristic and DL models are most efficient for detecting flooded areas and estimate water depth.
Crossdisciplinary collaboration
The researchers in the team represent KTH Royal Institute of Technology and Stockholm University.
About the project
Objective
We propose to use hybrid testing to innovate data-driven solutions for cardiac assistance. The project aims to enable data-driven evaluation of novel cardiac support devices to allow a rich and healthy life for patients with cardiovascular disease.
Background
We are currently witnessing an epidemic of heart failure with a rising incidence in the general population worldwide (2–7%) and a mean survival of only five years. The last decade has seen tremendous advances in device-based treatment options. However, progress has stalled, with only one device being currently approved for use in humans.
At the same time, novel hybrid mock circulation loops have been developed, allowing physical device interaction with a digital model of the human cardiovascular system. Here at KTH, we built Sweden’s first cardiovascular hybrid mock circulation. In this way, we can mimic unprecedented amounts of virtual and physical implantations of potential candidates of cardiac assistive technologies. The hope is that machine learning approaches can aid in identifying the ideal position and actuation profile of the cardiac assist device of the future.
Crossdisciplinary collaboration
The researchers in the team represent the KTH School of Electrical Engineering and Computer Science and the School of Chemistry, Biotechnology, and Health.
Watch the recorded presentation at the Digitalize in Stockholm 2023 event:
About the project
Objective
The main purpose of the DeepAqua project is to quantify the changes in surface water over time. We want to create a real-time monitoring system of changes in water bodies by combining remote sensing technologies, including optical and radar imagery, with deep learning techniques to perform computer vision and transfer learning. Employing this innovative strategy will allow us to calculate the water extent and level dynamics with unprecedented accuracy and response time speed. This approach offers a practical solution for monitoring water extent and level dynamics, making it highly adaptable and scalable for water conservation efforts.
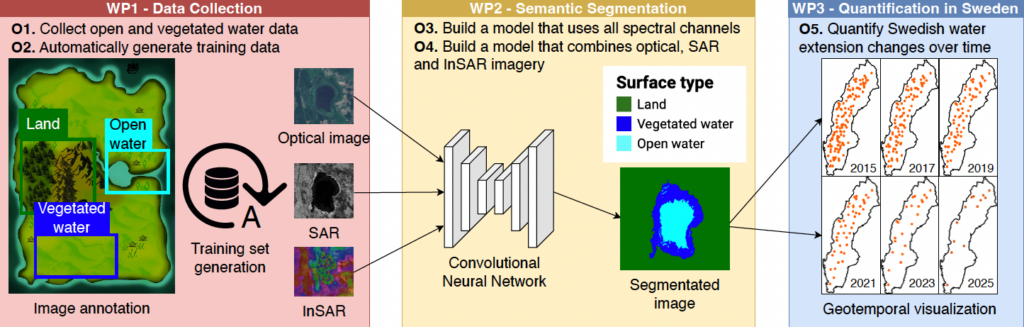
Background
Climate change presents one of the most formidable challenges to humanity. In the current year, we have witnessed unprecedented heatwaves, extreme floods, an increasing scarcity of water in various regions, and a troubling surge in the global extinction of species. Halting the advance of climate change necessitates the preservation of our existing water resources. However, recent advancements in remote sensing technology have yielded a wealth of high-quality data, opening up new avenues for researchers to leverage deep learning (DL) techniques in water detection. DL is a machine learning methodology that consistently outperforms traditional approaches across diverse domains, including computer vision, object recognition, machine translation, and audio processing.
This project, named DeepAqua, seeks to enhance our understanding of surface water dynamics and their response to environmental changes by developing innovative DL architectures, such as Convolutional Neural Networks (CNN) and Transformers, designed specifically for the semantic segmentation of water-related images. It is worth noting that many DL models depend on substantial amounts of ground truth data, which can be costly to obtain. Our previous findings suggest that we can train a CNN using water masks based on the Normalized Difference Water Index (NDWI) to detect water in Synthetic Aperture Radar (SAR) imagery without the need for manual annotation. This breakthrough promises to have a significant impact on water monitoring since generating data based on NDWI masks is virtually cost-free compared to traditional methods involving fieldwork data collection and manual annotation.
Crossdisciplinary collaboration
The researchers in the team represent the Division for Water and Environmental Engineering (SEED/ABE), the Division of Software and Computer Systems (CS/EECS), KTH, and Stockholm University.
About the project
Objective
This project aims to establish an AI-based online platform for automated, and robust personalization and positioning of HBMs, focusing on baby HBMs. By this we eliminate the need for users to tackle personalization and positioning which is often challenging and tedious, thus the platform could be an tranformative tool for driving innovations relating to HBMs.
Background
Finite element HBMs are digitalized representations of the human body and have emerged as significant tools for driving industrial innovation and clinical applications. These models often are a baseline and in a specified position, and before the use of the HBMs, personalization and positioning of HBMs are needed. Despite continuous active development, HBM positioning remains challenging and tedious.
Crossdisciplinary collaboration
This project brings expertise within biomechanical modeling and artificial intelligence involving researchers from KTH School of Electrical Engineering and Computer Science and Applied AI at the Department of Industrial Systems at Research Institutes of Sweden (RISE).
Former project name: Virtual Baby Plattform
About the project
Objective
In collaboration with Karolinska University Hospital (KUH) and Karolinska Institute (KI), the PI and Co-PI of KTH propose the ISPP postdoc project EMERDENSY to develop trust-worthy machine learning algorithms with explainable outcomes and then use the algorithms for the design of Early Warning Systems (EWS).
Background
Artificial Intelligence (AI) can be used to detect infection. Often, doctors and nurses cannot be sure about the growth of infection due to the absence of clearly visible symptoms. Once infection starts, the body’s immune system starts to fight bacteria and viruses. Physiological parameters of the body, such as heart rate, blood pressure, breathing patterns, and temperature, change slowly.
AI can detect subtle changes, but humans cannot. AI can also predict infection type and patient deterioration. The medical care team will then spend precious time deciding on life-saving interventions. This heralds the use of AI-based early warning systems (EWS).
The big question: can we trust the AI systems, mainly its core called machine learning for data analysis and predictions? Can the machine learning algorithms explain their predictions to the healthcare personnel?
Partner Postdoc(s)
Yogesh Todarwal
Main supervisor
Saikat Chatterjee, Associate Professor, Division of Information Science and Engineering at KTH
Co-supervisor
Sebastiaan Meijer, Professor and Vice Dean, Division of Health Informatics and Logistics at KTH
Watch the recorded presentation at the Digitalize in Stockholm 2023 event: