Date and time: Monday 9 June 2025, 15:00 – 16:00 CEST
Speaker: Yanina Shkel, EPFL
Title: On Binary Maximal Leakage and Interpreting Privacy Measures through Hypothesis Testing
Where: Digital Futures hub, Osquars Backe 5, floor 2 at KTH main campus OR Zoom
Directions: https://www.digitalfutures.kth.se/contact/how-to-get-here/
OR Zoom: https://kth-se.zoom.us/j/69560887455
Host: Tobias Oechtering, oech@kth.se
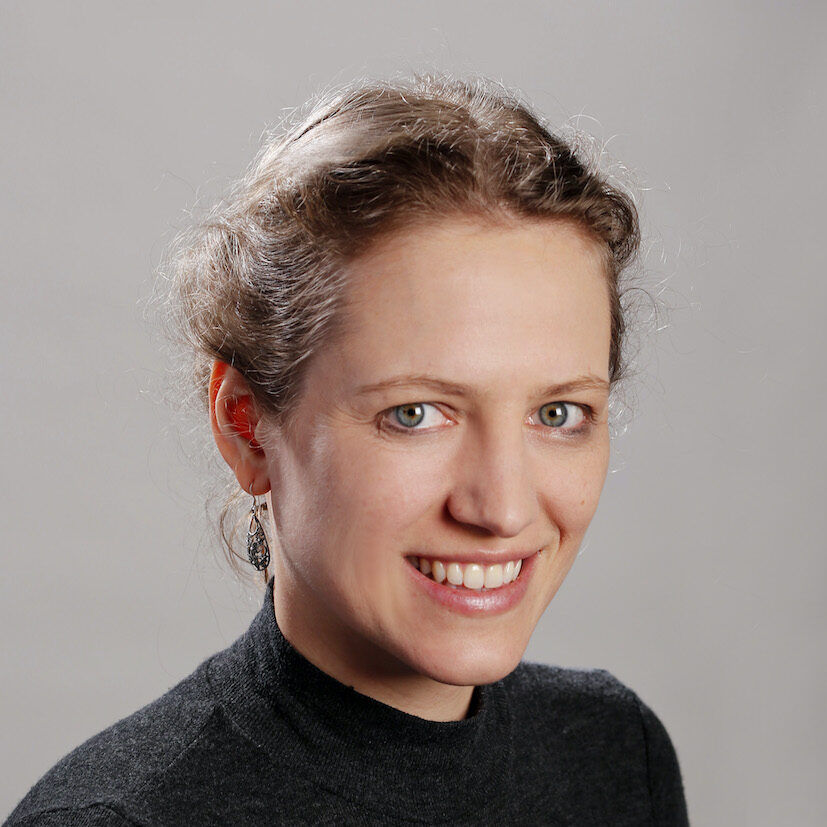
Bio: Yanina Shkel is an Assistant Professor with École Polytechnique Fédérale de Lausanne, where she is affiliated with the Information Processing Group in the School of Computer and Communication Sciences. She received the B.S. degree in mathematics and computer science and the M.S. and Ph.D. degrees in electrical and computer engineering from the University of Wisconsin–Madison. Before graduate school, she was a Database Developer with Morningstar Inc. During graduate school, she spent her time with 3M Corporate Research, as an Intern.
After completing her PhD in 2014, she was a Post-Doctoral Researcher with Princeton University and University of Illinois at Urbana–Champaign. She joined EPFL as a Scientist in 2019, and as an Assistant Professor in 2023. Her research interests include theoretical aspects of data science: in particular, statistics, cryptography, as well as information, learning, and coding theory. She was a recipient of the 2015 NSF Center for Science of Information (CSoI) Post-Doctoral Fellowship and the 2022 Swiss NSF Starting Grant.
Abstract: Given two random variables X and Y, the maximal leakage from X to Y was recently proposed as an operational privacy measure. Maximal leakage quantifies the multiplicative increase of the probability of correctly guessing any randomized function of X after observing Y. In this talk we focus on the properties of maximal leakage in the situation where only certain functions of X are assumed to be of interest to the adversary. We particularly focus on measuring maximal leakage with respect to all binary functions of X. We derive a computable form for this binary maximal leakage and characterize the class of dominating mechanisms. We also show that it satisfies standard properties, such as composition theorems and the data processing inequalities.
As it turns out, binary maximal leakage is equivalent to several other privacy measures proposed in the literature such as total variation privacy and Bayes security. We discuss a number of other interesting extensions for maximal leakage such as k-ary leakage and statistical maximal leakage. Finally, we review the hypothesis testing framework for differential privacy and how it relates to our new privacy measures.