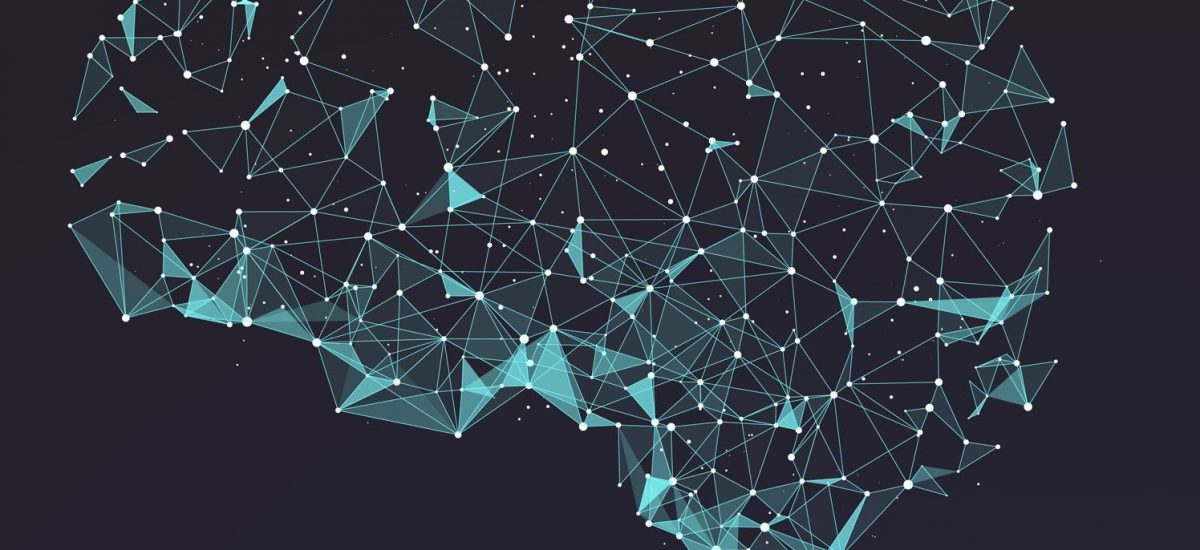
BrainNet workshop – Day 1
Date and time: 23 May 2022, 09:00 – 17:00 CEST (UTC +2)
Title: BrainNet workshop
Where: Digital Futures hub, Osquars Backe 5, floor 2 at KTH main campus
Directions: https://www.digitalfutures.kth.se/contact/how-to-get-here/
Maximum 50 participants are onsite at the Digital Futures hub. First-come, first-served basis.
For participation – please register here: https://www.kth.se/form/621dcfad3d24bd99b9be1b9c
For online participation, a Zoom link will be provided to registered participants closer to the event.
RSVP: 16 May
For whom
This hybrid workshop is aimed to appeal to researchers sharing an interest in network science, neuroscience, analysis of neural signals, and mathematical tools pertaining to these areas. The neuroscientific interest lies particularly in the diagnosis and prognosis of neurodegenerative diseases. The workshop days will take place in an inspiring environment and will be scheduled with ample time for discussion, thus maximizing the prospects of collaboration.
Information about the event
Network science has long been employed by neuroscience in questions ranging from microscopic networks of neurons to macroscopic networks of whole brain regions. How the neural network structure interacts with its dynamics, and how the brain processes information, remains an open question, due to the complexity and variability of neural activity and neural architectures. Likewise, inferring information from brain signals obtained by MEG and FMRI for the diagnosis and prognosis of, for example, neurodegenerative diseases is open for further development of tools. Rather recently, the above questions have also led to new developments in which highly sophisticated theoretical mathematics has been used to build new tools for neural data analysis, for example, the field of topological data analysis. Such developments need the involvement of both experimentalists and theoreticians for them to lead to explainable outputs. Our workshop will be a platform to establish new connections between people sharing an interest in any of the above fields.
The workshop is funded by Life Science Platform.
Questions? For more information please contact Henri Riihimäki: henrir@kth.se
Program
Monday 23 May
09:00 Arvind Kumar, KTH
Computational approaches to understand brain diseases
09:30 Travis Thompson, University of Oxford
Networking Neurodegeneration
10:15 Break
10:20 Keynote 1: Marja-Leena Linne, Tampere University (online talk)
Modelling Neurons, Astrocytes and Synaptic Plasticity Mechanisms to Understand Network Structure and Activity Dynamics in vitro and in vivo
11:05 Break
11:15 Keynote 2: Bastian Rieck, Helmholtz-Zentrum München (online talk)
A Gentle Introduction to Topological Machine Learning
12:00 – 13:00 Lunch
13:00 Ginestra Bianconi, Queen Mary University of London (online talk)
The dynamics of higher-order networks: the effect of topology and triadic interactions
13:45 Break
13:50 Giovanni Petri, ISI Foundation
Between higher-order mechanisms and phenomena
14:35 Coffee break
15:00 Ilaria Carannante, KTH
Exploring striatum in health and disease using data-driven models
15:45 Break
15:50 Florian Unger, TU Graz
Justifying TDA in Neuroscience: Efficient null-model generation by uniformly sampling directed graphs with similar simplex counts as connectomes
16:35 End of Day 1
Tuesday 24 May
09:30 Bastian Rieck, Helmholtz-Zentrum München
A Good Scale is Hard To Find: Shape Analysis Using Topology
10:15 Break
10:20 Jason Smith, University of Nottingham-Trent
To be announced
11:05 Break
11:15 Giorgio Nicoletti, University of Padova
What can criticality and phase transitions teach us about brain dynamics?
12:00 End of Day 2
Abstracts
Arvind Kumar
Computational approaches to understand brain diseases
Abstract: In my talk I will outline dynamical systems and information processing perspective to understand brain diseases. I will argue that theory of biological networks (both structure and dynamics) is essential to identify causal links between biomarkers of the diseases and behavioral symptoms. Finally, I will give an overview of how dBRAIN initiative is using these approaches to better understand neurodegenerative diseases.
Travis Thompson
Networking Neurodegeneration
Abstract: Can mathematics understand Alzheimer’s disease? The modern medical perspective on neurological diseases has evolved, slowly, since the 20th century but recent breakthroughs in medical imaging have quickly transformed medicine into a quantitative science. Today, mathematical modeling and scientific computing allow us to go farther than observation alone; data-informed mathematical models, that exploit the brain’s network structure, are leading to new clinical insights into neurodegenerative diseases such as Alzheimer’s disease. In this talk, I will introduce the fundamentals of neurodegenerative diseases and show how the network connectivity of the brain can be exploited to model their etiology and progression, match to clinical data and to gain insight into how animal experiments can shed light on disease progression in humans. In the end, we will see that network mathematical models are an indispensible tool for cultivating a data-informed understanding of neurodegenerative disease of the human brain.
Iris Yoon
Neural encoding and propagation with the semantics of persistent homology
Abstract: A central challenge in topological data analysis is the interpretation of barcodes. The classical approach is to build an explicit map to a well-understood model or a space and to leverage functoriality. However, we often lack such maps in real data. I will describe one possible way of addressing this issue that uses cross-system dissimilarity between the observations and the reference system. I will then share some preliminary results of using this method to study neural encoding and propagation on real & simulated data. This is joint work with Chad Giusti and Robert Ghrist, among others.
Bastian Rieck
Lecture on Topology & More: A Gentle Introduction to Topological Machine Learning
Abstract: Topology-based methods have started to emerge as a novel paradigm for data analysis tasks. Their stability with respect to noise, a consequence of their focus on fundamental properties of data, has made them attractive in numerous contexts. However, their integration into modern machine learning methods such as deep neural networks still proves challenging. Moreover, practitioners often find existing literature to be lacking when it comes to providing an intuitive description of topological concepts. This talk, aimed at a general audience of researchers, provides a gentle introduction to topology, with a specific eye towards applications in healthcare. I will prioritise building intuition and showcasing the most important concepts in this exciting nascent area.
Methods Talk on Recent Research: A Good Scale is Hard To Find: Shape Analysis Using Topology
Abstract: Our world is full of phenomena that happen at different spatial and temporal scales. If we pick the wrong scale, we might miss the forest for the trees – and vice versa! In recent years, methods from computational topology have started to emerge as one way to address the challenging task of picking the ‘right’ scale: Instead of enforcing one specific scale when analysing data, such methods afford an analysis of *all* scales inherent to data sets. In this talk, I will outline the general utility and expressivity of topological machine learning methods, i.e. methods combining a rigorous mathematical underpinning with the flexibility of modern deep learning architectures. I will provide both a theoretical as well as an applied view on this topic by showcasing how to employ topological methods to solve inverse problems, dealing with image reconstruction tasks in fluorescence microscopy.
Ginestra Bianconi
The dynamics of higher-order networks: the effect of topology and triadic interactions
Abstract: Higher-order networks capture the interactions among two or more nodes and they are raising increasing interest in the study of brain networks. Here we show that higher-order interactions are responsible for new dynamical processes that cannot be observed in pairwise networks. We will cover how topology is key to define synchronization of topological signals, i.e. dynamical signals defined not only on nodes but also on links, triangles and higher-dimensional simplices in simplicial complexes. Interesting topological synchronization dictated by the Dirac operator can lead to the spontaneous emergence of a rhythmic phase where the synchronization order parameter displays low frequency oscillations which might shed light on possible topological mechanisms for the emergence of brain rhythms. We will also reveal how triadic interactions can turn percolation into a fully-fledged dynamical process in which nodes can turn on and off intermittently in a periodic fashion or even chaotically leading to period doubling and a route to chaos of the percolation order parameter.
Ilaria Carannante
Exploring striatum in health and disease using data-driven models
Abstract: Simulating large-scale networks of neurons is an important approach when interpreting and synthesising different types of experimental data from the healthy as well as from the diseased brain. I will describe an open source pipeline for predicting microcircuit connectivity and for setting up simulations in a reproducible way (Hjorth et al., 2021). The brain area I will focus on is the striatum, the main input stage and largest nucleus of the basal ganglia. Basal ganglia are involved in motor learning, action-selection and reinforcement learning. Their dysfunction leads to a variety of brain disorders like Parkinson’s diseases (PD), a degenerative, progressive disorder that affects nerve cells. A nearly full-scale digital reconstruction of the mouse striatal microcircuitry (Hjorth, Kozlov et al., 2020) is used for the structural analysis, and then the results are compared when using neuron morphologies representing different stages of PD. Applications of graph theory together with simulations have already provided insights into the structural-functional connectivity of cortical networks (Reimann et al., 2017). I will show how we are applying algebraic topology, in particular directed cliques (simplices), to investigate the local structural connectivity in striatum, and how structural features shape network dynamics.
Giovanni Petri
Between higher-order mechanisms and phenomena
Abstract: Complex networks have become the main paradigm for modelling the dynamics of complex interacting systems. However, networks are intrinsically limited to describing pairwise interactions, whereas real-world systems are often characterized by higher-order interactions involving groups of three or more units. Higher-order structures, such as hypergraphs and simplicial complexes, are therefore a better tool to map the real organization of many social, biological and man-made systems. At the same time, higher-order observables, typically topological or information-theoretic in nature and often sharing the same simplicial language, have been gathering attention for their capacity to capture properties of complex systems that are invisible to standard statistical descriptions. This had led to a certain confusion between these two facets, mechanisms on one side, phenomena on the other. Here, using recent examples from both computational modeling and neuroimaging analysis, I highlight collective behaviours induced by higher-order interactions, their interface with recent advances in topological data analysis, and finally outline three key challenges for the physics of higher-order complex systems.
Giorgio Nicoletti
What can criticality and phase transitions teach us about brain dynamics?
Abstract: In the last twenty years, the idea that brain dynamics might be described by models close to a phase transition has emerged as an important and compelling framework. This hypothesis was first formulated in the seminal work of Beggs and Plenz, who showed that spontaneous neural activity displays a seemingly scale-free spatiotemporal organization, a feature typical of critical systems. In this talk, I will introduce the key ideas behind phase transitions and criticality, and why some of their properties might be desirable for neuronal networks. I will then review which signatures of criticality are typically found in data, and some possible interpretations in terms of effective and paradigmatic models. In particular, I will show how phase transitions appear in simple stochastic models, and how they give rise to emergent oscillations when these models are embedded in real brain networks. Finally, I will briefly illustrate how recent developments inspired by Statistical Physics can help unravel the collective properties of networks of neurons.
Florian Unger
Justifying TDA in Neuroscience: Efficient null-model generation by uniformly sampling directed graphs with similar simplex counts as connectomes
Abstract: Recent works in Topological Data Analysis have analyzed biological neural networks by understanding them as directed graphs and analyzing their flag complex. The significance of their findings was always with respect to comparable Erdös-Renyi-Graphs (ER-Graphs), despite them lacking a large enough flag complex to support more complex topological structures to begin with. This raises the question: Are these topological findings just a byproduct of the large number of simplices? We propose a different null-model than ER-Graphs of comparable size and density: We require additionally a comparable number of simplices in its flag complex. In this talk we’ll present a first step towards that: Whilst also retaining the underlying undirected graph, we develop a Monte-Carlo-Markov-Chain-based sampling algorithm able to uniformly sample from our proposed null-model. As a first result, we present that the connectome of C.Elegans does not only give rise to a higher number of simplices when compared to comparable ER-graphs (already known), but additionally has more topological features not only compared to an ER-graph, but also compared to our (connectivity-restrained) null-model. This suggests significance and purpose of topological methods beyond simplex count analysis.”
Marja-Leena Linne
Modeling Neurons, Astrocytes and Synaptic Plasticity Mechanisms to Understand Network Structure and Activity Dynamics in vitro and in vivo
Abstract: to be announced