Date and time: Monday 2 June 2025, 11:00-12:00 CEST
Speaker: Maurizio Filippone – KAUST
Title: Priors for Bayesian Deep Learning
Where: VIC-studion, Lindstedtsvägen 9
Directions: https://www.kth.se/places/room/id/ae9e43c4-cb44-4d47-af73-895d2a8c24c6
OR
Zoom: https://kth-se.zoom.us/j/67030594606
Host: Jens Lagergren, jensl@kth.se
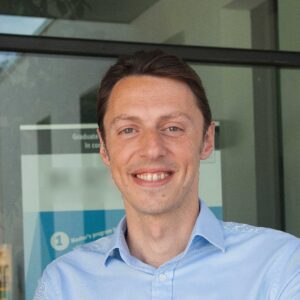
Bio: Maurizio Filippone received a Master’s degree in Physics and a Ph.D. in Computer Science from the University of Genova, Italy, in 2004 and 2008, respectively. In 2007, during his Ph.D. studies, he visited George Mason University, Fairfax, VA as a Research Scholar. From 2008 to 2011, he was a Research Associate with the University of Sheffield, U.K. (2008-2009), with the University of Glasgow, U.K. (2010), and with University College London, U.K (2011). In 2011, he took up a Lecturer position at the University of Glasgow, U.K, which he left in 2015 to join EURECOM, Sophia Antipolis, France as an Associate Professor.
In 2024 he joined the Statistics Program at KAUST as an Associate Professor. His current research interests include the development of tractable and scalable Bayesian inference techniques for Gaussian processes and Deep Learning models with applications in life and environmental sciences.
Abstract: The impressive success of Deep Learning (DL) in predictive performance tasks has fueled the hopes that this can help addressing societal challenges by supporting sound decision making. However, many open questions remain about their suitability to hold up to this promise. In this talk, I will discuss some of the current limitations of DL, which directly affect their wide adoption.
I will focus in particular on the poor ability of DL models to quantify uncertainty in predictions, and I will present Bayesian DL as an attractive approach combining the flexibility of DL with probabilistic reasoning. I will then discuss the challenges associated with carrying out inference and specifying sensible priors for DL models. After presenting some recent contributions to address these problems, I will conclude by presenting some interesting emerging research trends and open problems.